Named entity recognition model training method and named entity recognition method and device
A technology for named entity recognition and model training, which is applied to instruments, electrical digital data processing, computing, etc., and can solve problems that affect the recognition effect, are not applicable, and model features rely on manual work.
- Summary
- Abstract
- Description
- Claims
- Application Information
AI Technical Summary
Problems solved by technology
Method used
Image
Examples
Embodiment 1
[0077] figure 1 It is a schematic flow chart of a named entity recognition model training method provided by an embodiment of the present invention, refer to figure 1 As shown, the method may include the steps of:
[0078] Step 101, preprocessing the corpus samples to obtain character sequence samples, and labeling the character sequence samples with named entity labels to obtain training character sequences.
[0079] Specifically, word segmentation is performed on the corpus samples to obtain multiple word segmentations, and all individual characters are decomposed from multiple word segmentations to obtain character sequence samples; the character sequence samples are labeled with corresponding named entity labels according to the BMEO labeling rules to obtain training characters sequence.
[0080] In this embodiment, an open source word segmentation tool (such as a stuttering word segmentation tool) can be used to perform word segmentation processing on the corpus samples...
Embodiment 2
[0124] Based on the named entity recognition model trained in Embodiment 1, the embodiment of the present invention also provides a named entity recognition method. After the named entity recognition model is deployed as a service, the named entity recognition method can realize the text to be marked Quickly call the online named entity recognition model for named entity recognition.
[0125] refer to figure 2 As shown, the embodiment of the present invention provides a named entity recognition method, the method comprising:
[0126] Step 201, preprocessing the text to be marked to obtain a sequence of characters to be marked.
[0127] Specifically, word segmentation processing is performed on the text to be labeled to obtain multiple word segmentations, and all individual characters are decomposed from the multiple word segmentations to obtain a sequence of characters to be labeled.
[0128] In this embodiment, an open source word segmentation tool (such as a stammering wo...
Embodiment 3
[0152] image 3 is a schematic structural diagram of a named entity recognition model training device provided by an embodiment of the present invention, as image 3 As shown, the device includes:
[0153] The training data acquisition module 31 is used to preprocess the corpus samples to obtain character sequence samples, and mark the character sequence samples with named entity labels to obtain training character sequences;
[0154] The first pre-training module 32 is used to pre-train the training character sequence based on the preset first two-way language model and the first self-attention mechanism model, and obtain a character feature vector and a character weight vector corresponding to the training character sequence;
[0155] The second pre-training module 33 is used to pre-train the training character sequence based on the preset second two-way language model and the second self-attention mechanism model, and obtain a word feature vector and a word weight vector c...
PUM
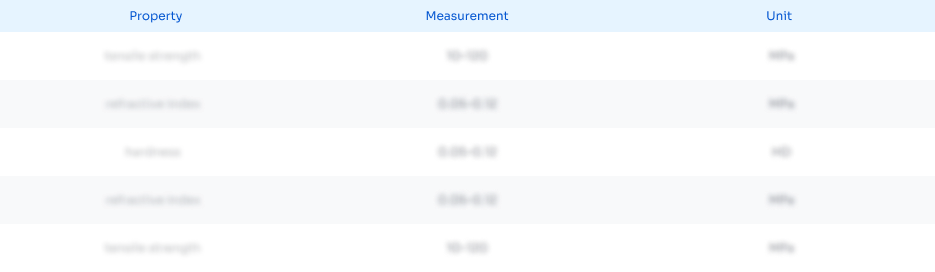
Abstract
Description
Claims
Application Information
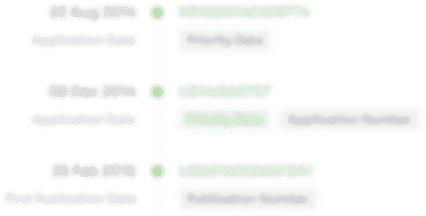
- R&D
- Intellectual Property
- Life Sciences
- Materials
- Tech Scout
- Unparalleled Data Quality
- Higher Quality Content
- 60% Fewer Hallucinations
Browse by: Latest US Patents, China's latest patents, Technical Efficacy Thesaurus, Application Domain, Technology Topic, Popular Technical Reports.
© 2025 PatSnap. All rights reserved.Legal|Privacy policy|Modern Slavery Act Transparency Statement|Sitemap|About US| Contact US: help@patsnap.com