Deep learning traffic classification method based on combination of time-space characteristics
A deep learning and traffic classification technology, applied in the field of computer networks, can solve the problems of destroying the end-to-end structure of deep learning, reducing the self-learning ability of deep learning, unable to classify network traffic, etc., to achieve good service experience and service quality, The effect of saving labor and time cost, good generalization ability and applicability
- Summary
- Abstract
- Description
- Claims
- Application Information
AI Technical Summary
Problems solved by technology
Method used
Image
Examples
Embodiment Construction
[0044] Embodiments of the present invention will be further described in detail below in conjunction with the accompanying drawings.
[0045] refer to figure 1 , the implementation steps of the embodiment are as follows.
[0046] Step 1. Collect and label original network traffic load data.
[0047] 1.1) Collect network traffic load data from pure network nodes, and classify them according to encrypted traffic, unencrypted traffic and malicious access traffic. The encrypted traffic is subdivided and marked according to six major types of applications in the Internet, namely Email , Chat, File, P2P, Streaming, and VoIP;
[0048] 1.2) Randomly mix the network traffic load data collected this time, the previous time point data and the pre-built database to expand the content of the database, reduce the blind spots after the deep learning model training, and obtain the marked network traffic load database.
[0049] Step 2: Generate a preprocessed traffic atlas based on the labe...
PUM
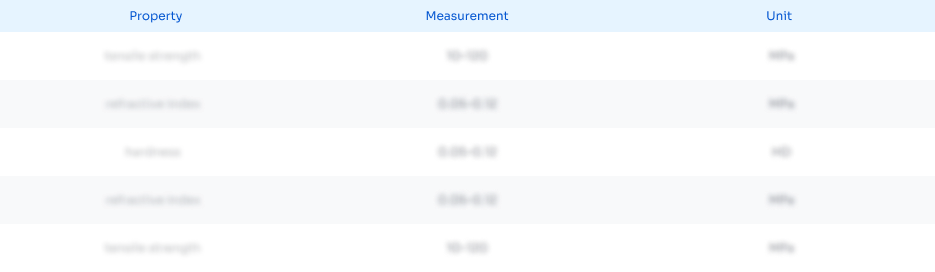
Abstract
Description
Claims
Application Information
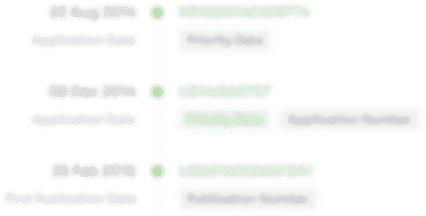
- R&D Engineer
- R&D Manager
- IP Professional
- Industry Leading Data Capabilities
- Powerful AI technology
- Patent DNA Extraction
Browse by: Latest US Patents, China's latest patents, Technical Efficacy Thesaurus, Application Domain, Technology Topic, Popular Technical Reports.
© 2024 PatSnap. All rights reserved.Legal|Privacy policy|Modern Slavery Act Transparency Statement|Sitemap|About US| Contact US: help@patsnap.com