Electronic equipment, task processing method and neural network training method
A neural network and task processing technology, applied in biological neural network models, neural architectures, neural learning methods, etc., can solve the problems of high computational cost and storage cost of neural networks, high task processing complexity, and reduce computational costs and storage costs. Cost and complexity reduction
- Summary
- Abstract
- Description
- Claims
- Application Information
AI Technical Summary
Problems solved by technology
Method used
Image
Examples
Embodiment 1
[0168] The embodiment of the present application provides a method for task processing based on a neural network. The method includes: obtaining input data corresponding to the task, and extracting feature information corresponding to each neural network base from the input data corresponding to the task, for example, neural network The feature information corresponding to network base 1, the feature information corresponding to neural network base 2...the feature information corresponding to neural network base n, and the extracted feature information is input to the corresponding neural network base, and then the output of each neural network base The results are weighted and summed based on the weight information of each neural network base corresponding to each subtask of the task or task, and the corresponding processing results of the task or subtask are obtained. For example, when the task contains m subtasks, the output results of each neural network base are based on S...
Embodiment 2
[0224] Another possible implementation of the embodiment of the present application, on the basis of the first embodiment, also includes the operations shown in the second embodiment, wherein,
[0225] This embodiment at least introduces the process of online adaptive learning (online update), wherein the process of online adaptive learning includes: updating the neural network base and / or updating the neural network weight information corresponding to the task , if the task contains subtasks, updating the neural network weight information corresponding to the task includes updating the neural network base weight information corresponding to at least one subtask, specifically as follows including step S406 (not marked in the figure), wherein step S406 (not marked in the figure) can be performed before any of step S401, step S402, step S403, step 404 and step S405, can also be performed after any of the steps, and can also be performed simultaneously with any of the steps.
[0...
Embodiment 3
[0273]This embodiment can be implemented on the basis of any one of Embodiments 1 to 2, and can also be implemented independently of the above embodiments. If this embodiment is implemented on the basis of Embodiments 1 to 2, then this embodiment Step S501-step S504 in the example can be carried out before step S401, as Figure 5a As shown, wherein, the operations performed by step S505-step S509 are similar to the operations performed by step S401-step S405, and will not be repeated here; The execution sequence is as follows Figure 5b As shown, among them,
[0274] Embodiment 3 is the process of training the neural network base offline, such as Figure 6 As shown, it mainly includes collecting training data, extracting features from the training data, training the constructed neural network base based on the extracted features, and then training the weight of the neural network base corresponding to the task, as follows:
[0275] Step S501, constructing each neural networ...
PUM
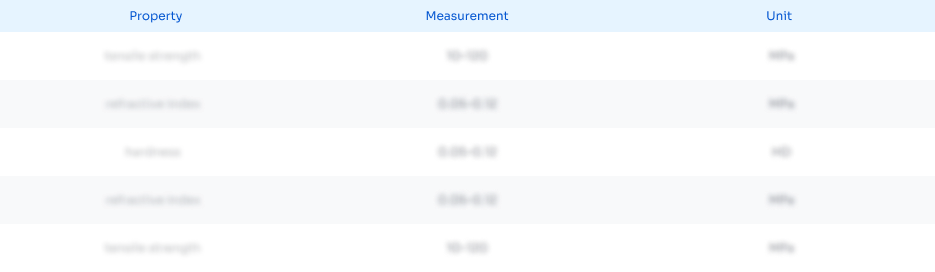
Abstract
Description
Claims
Application Information
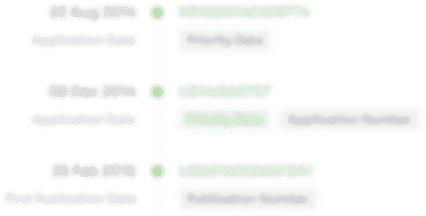
- R&D Engineer
- R&D Manager
- IP Professional
- Industry Leading Data Capabilities
- Powerful AI technology
- Patent DNA Extraction
Browse by: Latest US Patents, China's latest patents, Technical Efficacy Thesaurus, Application Domain, Technology Topic, Popular Technical Reports.
© 2024 PatSnap. All rights reserved.Legal|Privacy policy|Modern Slavery Act Transparency Statement|Sitemap|About US| Contact US: help@patsnap.com