Semi-supervised network representation learning model based on hierarchical attention mechanism
A network representation and learning model technology, applied in special data processing applications, unstructured text data retrieval, semantic analysis, etc., can solve problems such as undiscovered patent documents
- Summary
- Abstract
- Description
- Claims
- Application Information
AI Technical Summary
Problems solved by technology
Method used
Image
Examples
Embodiment Construction
[0087] The present invention will be further described in detail below through the specific examples, the following examples are only descriptive, not restrictive, and cannot limit the protection scope of the present invention with this.
[0088] The present invention mainly adopts the theory and method related to natural language processing and network representation learning to carry out representation learning on paper citation network data. In order to ensure the training and testing of models, the computer platform used is required to be equipped with no less than 8G of memory, and the number of CPU cores Not less than 4, and install Python3.6 version, tensorflow framework and other necessary programming environments.
[0089] Such as figure 2 As shown, the semi-supervised network representation learning method based on the hierarchical attention mechanism provided by the present invention includes the following steps performed in sequence:
[0090] Step 1) Input the te...
PUM
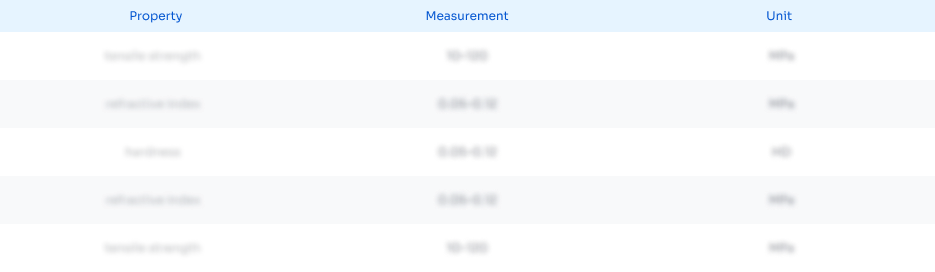
Abstract
Description
Claims
Application Information
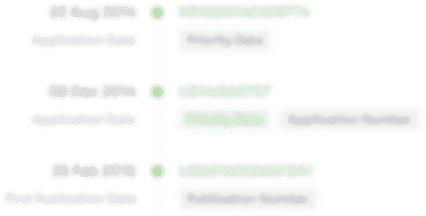
- R&D Engineer
- R&D Manager
- IP Professional
- Industry Leading Data Capabilities
- Powerful AI technology
- Patent DNA Extraction
Browse by: Latest US Patents, China's latest patents, Technical Efficacy Thesaurus, Application Domain, Technology Topic, Popular Technical Reports.
© 2024 PatSnap. All rights reserved.Legal|Privacy policy|Modern Slavery Act Transparency Statement|Sitemap|About US| Contact US: help@patsnap.com