Visual analysis method for understanding graph convolutional neural network
A technology of convolutional neural network and analysis method, which is applied in the direction of neural learning method, biological neural network model, neural architecture, etc., and can solve problems affecting the prediction accuracy of GCN model
- Summary
- Abstract
- Description
- Claims
- Application Information
AI Technical Summary
Problems solved by technology
Method used
Image
Examples
Embodiment Construction
[0052] The present invention will be specifically introduced below in conjunction with the accompanying drawings and specific embodiments.
[0053] Such as figure 1 As shown, a visual analysis method for understanding graph convolutional neural networks includes the following steps:
[0054] Step 1, divide the input graph structure data set into training set, verification set and test set;
[0055] Step 2, define a parameter set, including a set of hidden layers and a set of hidden neurons;
[0056] Step 3, based on the defined parameter set, train a series of graph convolutional neural network models;
[0057] Step 4, design the hidden layer analysis view to show the influence of hidden layer parameters on classification accuracy;
[0058] Step 5, design the loss and accuracy view to show the change of loss and classification accuracy during the iterative training process of the model;
[0059] Step 6: Use the GraphTSNE visualization method to calculate the position of th...
PUM
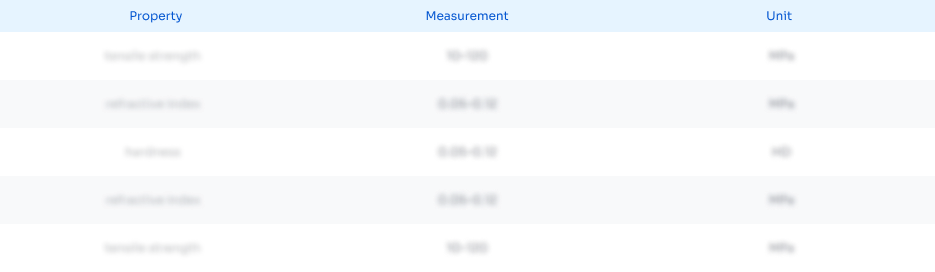
Abstract
Description
Claims
Application Information
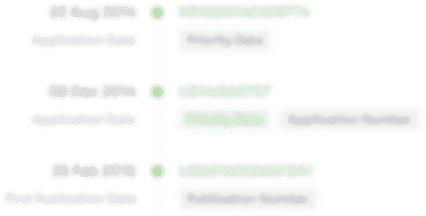
- Generate Ideas
- Intellectual Property
- Life Sciences
- Materials
- Tech Scout
- Unparalleled Data Quality
- Higher Quality Content
- 60% Fewer Hallucinations
Browse by: Latest US Patents, China's latest patents, Technical Efficacy Thesaurus, Application Domain, Technology Topic, Popular Technical Reports.
© 2025 PatSnap. All rights reserved.Legal|Privacy policy|Modern Slavery Act Transparency Statement|Sitemap|About US| Contact US: help@patsnap.com