Weld surface defect identification method based on image texture
A technology for weld surface and defect identification, which is applied in the field of non-destructive testing and can solve problems such as not being applied to welds.
- Summary
- Abstract
- Description
- Claims
- Application Information
AI Technical Summary
Problems solved by technology
Method used
Image
Examples
Embodiment Construction
[0068] Below in conjunction with concrete experiment the present invention will be further described:
[0069] The implementation process of this experiment includes the following steps:
[0070] In the experiment, five types of images with different surface welding quality (good, hole, welding deviation, welding bump and broken welding) were selected, and a total of 5×50 images were used as training samples. In addition, 5×8 images are collected as test samples.
[0071] Step 1: Image acquisition.
[0072] Image acquisition is performed on welds with different surface welding quality. In order to ensure the clarity of the image, errors caused by external light are corrected. Before collecting, set the camera's white balance red to 112, white balance green to 62, white balance blue to 123, hue to 15, saturation to 130 and contrast to -4. The collected images are true color RGB images. (Welds with different welding qualities are shown in Figure 1)
[0073] Step 2: Image pr...
PUM
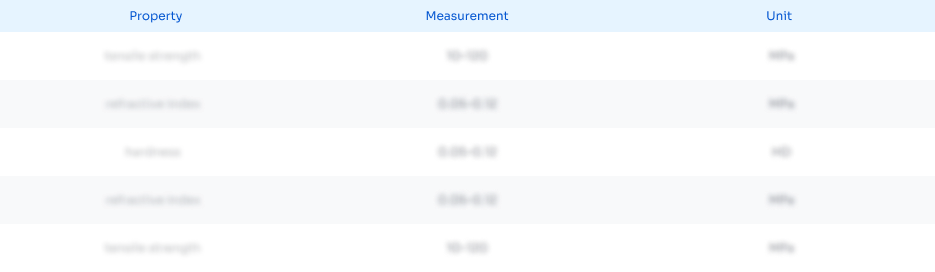
Abstract
Description
Claims
Application Information
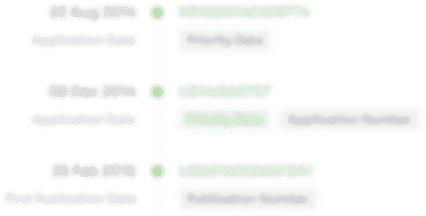
- R&D Engineer
- R&D Manager
- IP Professional
- Industry Leading Data Capabilities
- Powerful AI technology
- Patent DNA Extraction
Browse by: Latest US Patents, China's latest patents, Technical Efficacy Thesaurus, Application Domain, Technology Topic, Popular Technical Reports.
© 2024 PatSnap. All rights reserved.Legal|Privacy policy|Modern Slavery Act Transparency Statement|Sitemap|About US| Contact US: help@patsnap.com