Efficient short video content intelligent classification method based on deep learning and attention mechanism
A classification method and deep learning technology, applied in the field of computer vision, to achieve the effect of taking into account the prediction accuracy, improving model performance, and improving prediction accuracy
- Summary
- Abstract
- Description
- Claims
- Application Information
AI Technical Summary
Problems solved by technology
Method used
Image
Examples
Embodiment Construction
[0029] The present invention will be further illustrated below in conjunction with specific implementation examples.
[0030] Such as figure 1 As shown, the overall framework of the network is a series connection of two convolutional neural networks, and finally outputs the predicted probability of each category.
[0031] Such as figure 2 As shown, the specific process is as follows: First, uniformly draw frames from the short video, here the number of extracted frame images is set to N: first divide all video frames into N shares, and then randomly extract a frame from each share, in chronological order Arranged and fed into a two-dimensional convolutional neural network. The attention mechanism adopts the Squeeze- and-excitation module.
[0032] Such as image 3 As shown, the details of the two-dimensional convolutional neural network layer are described, including the detailed network structure diagram and convolution kernel parameters. Here, the two-dimensional convo...
PUM
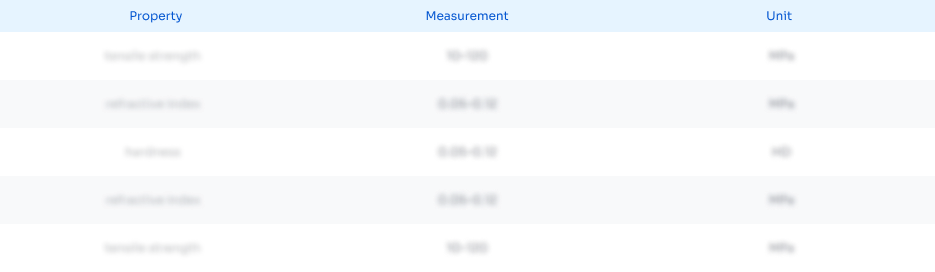
Abstract
Description
Claims
Application Information
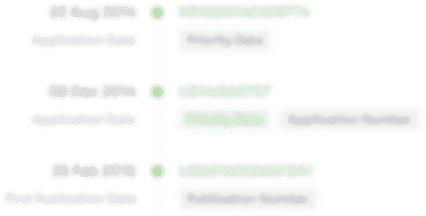
- R&D
- Intellectual Property
- Life Sciences
- Materials
- Tech Scout
- Unparalleled Data Quality
- Higher Quality Content
- 60% Fewer Hallucinations
Browse by: Latest US Patents, China's latest patents, Technical Efficacy Thesaurus, Application Domain, Technology Topic, Popular Technical Reports.
© 2025 PatSnap. All rights reserved.Legal|Privacy policy|Modern Slavery Act Transparency Statement|Sitemap|About US| Contact US: help@patsnap.com