Malicious code detection method based on API fragments
A malicious code detection and malicious code technology, applied in biological neural network models, instruments, platform integrity maintenance, etc., can solve problems such as difficult to deal with malicious code variants, limited malicious code detection capabilities, etc., to achieve anti-malicious code camouflage technology, Improved detection capability and high adaptability
- Summary
- Abstract
- Description
- Claims
- Application Information
AI Technical Summary
Problems solved by technology
Method used
Image
Examples
Embodiment 1
[0031] Firstly, the partial maliciousness of the malicious code is analyzed, taking the Trojan horse as an example, using the IDA Pro tool for reverse analysis, as shown in figure 1 As shown, it has been verified that the maliciousness is mainly reflected in a group of malicious API continuous calls, that is, an API fragment constitutes the privilege escalation operation of the Trojan horse. Further analysis found that the maliciousness of the malicious code is mainly reflected in the call of the malicious API, and usually the call of the malicious API can only form a malicious operation if it is executed sequentially by a group of APIs. At the same time, this kind of maliciousness is also based on locality, that is, local maliciousness, such as figure 2 as shown,
[0032] In the API execution sequence of a malicious code sample, most of the API sequences are normal, and only a few API fragments are malicious. Therefore, whether the code is malicious can be determined by ju...
PUM
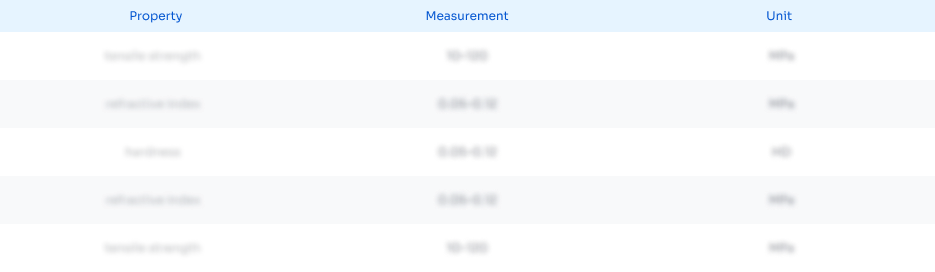
Abstract
Description
Claims
Application Information
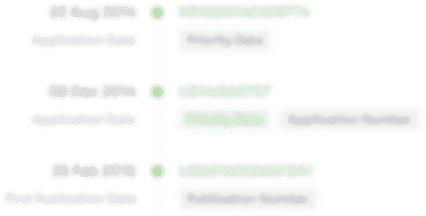
- R&D Engineer
- R&D Manager
- IP Professional
- Industry Leading Data Capabilities
- Powerful AI technology
- Patent DNA Extraction
Browse by: Latest US Patents, China's latest patents, Technical Efficacy Thesaurus, Application Domain, Technology Topic, Popular Technical Reports.
© 2024 PatSnap. All rights reserved.Legal|Privacy policy|Modern Slavery Act Transparency Statement|Sitemap|About US| Contact US: help@patsnap.com