Piano music score difficulty identification method based on lifting decision tree
A recognition method and decision tree technology, applied in character and pattern recognition, instruments, computer parts, etc., can solve problems such as inability to use difficulty level label classification, and achieve the effect of strong stability
- Summary
- Abstract
- Description
- Claims
- Application Information
AI Technical Summary
Problems solved by technology
Method used
Image
Examples
Embodiment Construction
[0030] The invention introduces a method for recognizing the difficulty of piano scores based on XGBoost (a learning algorithm based on boosting decision trees). The present invention is based on two data sets with larger data volumes. Compared with SVM and KNN, the XGBoost algorithm is an integrated learning algorithm with stronger classification performance, and supports parallel computing, and can choose a suitable logarithmic loss function. At the same time, the XGBoost algorithm involves multiple parameters that control the complexity of the model, and the model can be optimized in more detail. The grid search algorithm is used to select the optimal parameters, and a multi-classification XGBoost model based on grid search is established. Finally, the test set is used to establish The model is checked for accuracy. The invention enables the recognition of the difficulty level of the piano score to obtain higher accuracy and stability, provides reliable piano difficulty inf...
PUM
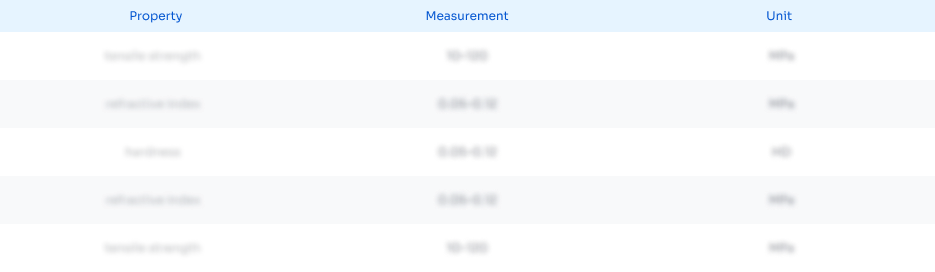
Abstract
Description
Claims
Application Information
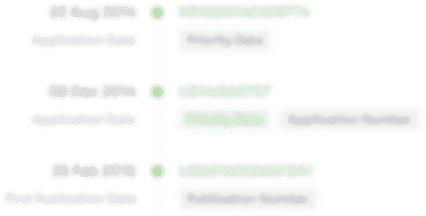
- R&D Engineer
- R&D Manager
- IP Professional
- Industry Leading Data Capabilities
- Powerful AI technology
- Patent DNA Extraction
Browse by: Latest US Patents, China's latest patents, Technical Efficacy Thesaurus, Application Domain, Technology Topic, Popular Technical Reports.
© 2024 PatSnap. All rights reserved.Legal|Privacy policy|Modern Slavery Act Transparency Statement|Sitemap|About US| Contact US: help@patsnap.com