A Construction Method of Multi-task Classification Network Based on Orthogonal Loss Function
A loss function and classification network technology, applied in neural learning methods, biological neural network models, neural architectures, etc., can solve problems such as lack of common features and unique feature distinctions
- Summary
- Abstract
- Description
- Claims
- Application Information
AI Technical Summary
Problems solved by technology
Method used
Image
Examples
Embodiment Construction
[0032] The invention is further illustrated below with reference to the accompanying drawings and examples.
[0033] Step a: Construction tag tree hierarchy;
[0034]Because there is a visual similarity between the species of the same race, the slab tree is constructed to construct a hierarchical label tree for a different database of species in nature. The structure of the hierarchical tag tree is divided into two layers, the first layer of label is the crude label of the image, according to the species classified, the second layer label is the sub-tag of the image, which is defined according to which species belongs to which species belongs. The branch of the label tree represents the dependency. Caltech-UCSD BIRDS-200-2011 is a bird database, a constructor is to divide crude clan in accordance with the subject of bird species by reviewing the encyclopedia knowledge. For example, the hummingbird is identified as a crude category of birds, which is a primary label of the sample. ...
PUM
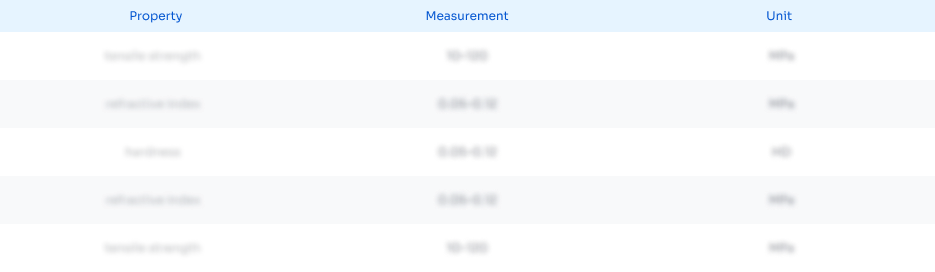
Abstract
Description
Claims
Application Information
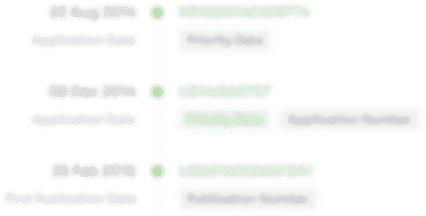
- R&D
- Intellectual Property
- Life Sciences
- Materials
- Tech Scout
- Unparalleled Data Quality
- Higher Quality Content
- 60% Fewer Hallucinations
Browse by: Latest US Patents, China's latest patents, Technical Efficacy Thesaurus, Application Domain, Technology Topic, Popular Technical Reports.
© 2025 PatSnap. All rights reserved.Legal|Privacy policy|Modern Slavery Act Transparency Statement|Sitemap|About US| Contact US: help@patsnap.com