Target detection method based on dense connection deep network
A dense connection, target detection technology, applied in neural learning methods, biological neural network models, instruments, etc., can solve problems such as increased network computing, limited network applications, large video memory, etc., to strengthen feature propagation and improve detection results. , the effect of enhanced learning
- Summary
- Abstract
- Description
- Claims
- Application Information
AI Technical Summary
Problems solved by technology
Method used
Image
Examples
Embodiment 1
[0052] Although the existing object detection methods have high detection accuracy, they cannot meet the requirements of real-time detection in actual production, and their portability is poor due to the model memory. In view of these problems, the present invention proposes a kind of target detection method based on densely connected deep network, which will be described in detail below in conjunction with the accompanying drawings:
[0053] Such as figure 1 As shown, it is a structural diagram of a densely connected network based on a densely connected deep network target detection method provided by the present invention; as figure 2 As shown, it is a network overall architecture diagram of a densely connected deep network-based object detection method provided by the present invention. In this embodiment, a target detection method based on a densely connected deep network includes the following parts:
[0054] A.1. Read the image data in the Pascal VOC dataset and extra...
Embodiment 2
[0079] This embodiment is the process and results of human detection on the Pascal VOC dataset. Specific steps are as follows:
[0080] A.1 Read in the pedestrian image data in different scenes in the Pascal VOC dataset as training data and extract pedestrian data features: the network reads the input image, first normalizes its resolution to 416*416, and then passes through the convolutional layer , pooling layer and dense connection module (Dense Block) extract and fuse the features of each channel.
[0081] Said step A.1 comprises:
[0082] (1) The dense connection method introduced makes the L-layer network have L(L+1) / 2 connections. The dense connection module Dense Block is mainly composed of 1*1 and 3*3 convolution layers, and the 1*1 convolution operation is also called bottleneck layer, the purpose is to reduce the number of input feature maps, improve computational efficiency and integrate characteristics of each channel. 3*3 convolution is used to extract image ...
Embodiment 3
[0107] This embodiment is the process and result of detecting the category of horses on the Pascal VOC dataset. Specific steps are as follows:
[0108] A.1 Read in the image data of horses in different scenes in the Pascal VOC dataset as training data and extract the characteristics of horse category data: the network reads the input image, first normalizes its resolution to 416*416, and then passes The convolutional layer, pooling layer, and dense connection module (Dense Block) extract and fuse the features of each channel.
[0109] Said step A.1 comprises:
[0110] (1) The dense connection method introduced makes the L-layer network have L(L+1) / 2 connections. The Dense Block is mainly composed of 1*1 and 3*3 convolutional layers, and the 1*1 convolution operation is also called the bottleneck layer. The purpose is to reduce the number of input feature maps, improve computational efficiency and integrate various channels. Characteristics. 3*3 convolution is used to extra...
PUM
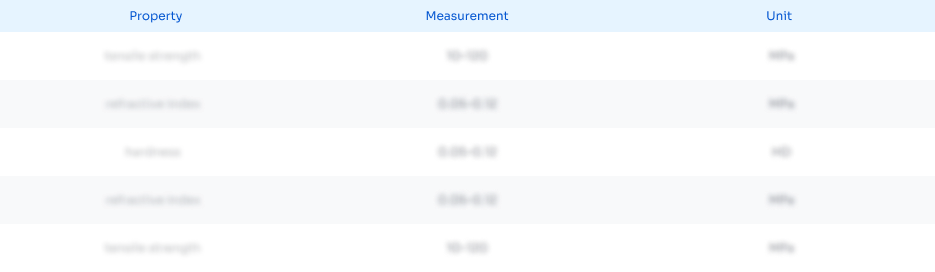
Abstract
Description
Claims
Application Information
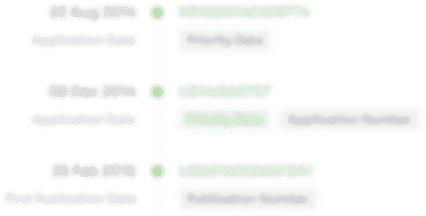
- Generate Ideas
- Intellectual Property
- Life Sciences
- Materials
- Tech Scout
- Unparalleled Data Quality
- Higher Quality Content
- 60% Fewer Hallucinations
Browse by: Latest US Patents, China's latest patents, Technical Efficacy Thesaurus, Application Domain, Technology Topic, Popular Technical Reports.
© 2025 PatSnap. All rights reserved.Legal|Privacy policy|Modern Slavery Act Transparency Statement|Sitemap|About US| Contact US: help@patsnap.com