Leukocyte nucleus and cytoplasm automatic segmentation method and system based on deep learning
A white blood cell nucleus, deep learning technology, applied in the field of medical image processing, can solve the problems of affecting the accuracy of the results, the classification accuracy needs to be improved, and the time-consuming, etc., to achieve a good segmentation effect and achieve the effect of automatic semantic segmentation.
- Summary
- Abstract
- Description
- Claims
- Application Information
AI Technical Summary
Problems solved by technology
Method used
Image
Examples
Embodiment 1
[0033] The present disclosure provides a deep learning-based method for automatically segmenting white blood cell nuclei and cytoplasm, including the following steps:
[0034] Step 1: Dataset selection and preprocessing.
[0035] The selected data set is the public data set of Jiangxi Taikang Technology Co., Ltd., in which the images are taken by the MoticMoticam Pro 252A optical microscope camera and the N800-D electric auto-focus microscope, including 300 white blood cell images of 224*224 pixels, including white blood cells The five categories are neutrophils, eosinophils, basophils, lymphocytes, and monocytes. Masked parts are annotated by experts.
[0036] The marked data is preprocessed, and the preprocessing includes grayscale normalization and fixed grayscale relabeling operations on the mask part. The background, white blood cell nucleus, and cytoplasm were rescaled to gray values of 0, 1, and 2, respectively; and the data set was divided into three parts: trainin...
Embodiment 2
[0060] The present disclosure provides an automatic segmentation system for white blood cell nuclei and cytoplasm based on deep learning, including:
[0061] The U-shaped neural network segmentation model building block is configured as a U-shaped neural network segmentation model including an encoder and a decoder, the encoder uses an improved neural network structure for feature extraction, and the decoder restores images by upsampling The details and spatial information of the encoder and the decoder are connected by jump connections to supplement the underlying information lost in the pooling process;
[0062] The training module is configured as a U-shaped neural network segmentation model constructed by using the white blood cell training set training. The loss of the white blood cell verification set is used as the monitoring index, and the learning rate is set and adjusted when the monitoring index remains unchanged to obtain the trained U-shaped neural network. Networ...
Embodiment 3
[0065] The present disclosure provides an electronic device, including a memory, a processor, and computer instructions stored in the memory and run on the processor. When the computer instructions are run by the processor, an automatic segmentation of white blood cell nucleus and cytoplasm based on deep learning is completed. steps described in the method.
PUM
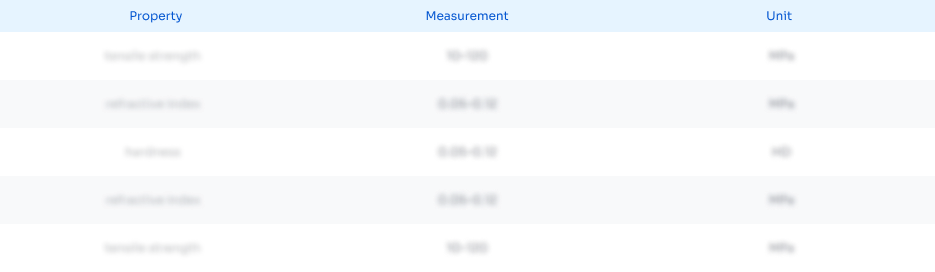
Abstract
Description
Claims
Application Information
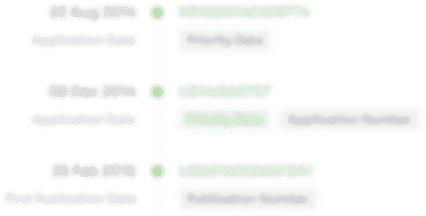
- R&D Engineer
- R&D Manager
- IP Professional
- Industry Leading Data Capabilities
- Powerful AI technology
- Patent DNA Extraction
Browse by: Latest US Patents, China's latest patents, Technical Efficacy Thesaurus, Application Domain, Technology Topic, Popular Technical Reports.
© 2024 PatSnap. All rights reserved.Legal|Privacy policy|Modern Slavery Act Transparency Statement|Sitemap|About US| Contact US: help@patsnap.com