Muskmelon quality detection method based on hyperspectral image depth feature fusion
A hyperspectral image and depth feature technology, applied in the field of melon quality detection based on the fusion of hyperspectral image depth features, can solve the problems of high complexity of deep network models, failure to integrate low-level information, and ignore edge information extraction, etc., to overcome the model The effect of the overfitting problem
- Summary
- Abstract
- Description
- Claims
- Application Information
AI Technical Summary
Problems solved by technology
Method used
Image
Examples
Embodiment 1
[0036] The embodiment of the present invention describes an end-to-end network structure based on deep multi-scale feature fusion, such as figure 1 shown.
[0037] It has two features: First, due to the end-to-end training strategy, all training processes are integrated into a neural network, which will make the learned features more discriminative. Second, unlike traditional frameworks that separate spectral and spatial features, spectral and spatial features are trained simultaneously in the proposed method. In this way, spectral and spatial information can be better fused. The main contributions of this method are summarized as follows.
[0038] 1. An end-to-end melon sweetness and firmness prediction framework (as an unsupervised network model) is proposed, which integrates the spectral and spatial features of melons into a unified neural network, which is divided into 2 branch:
[0039] The first branch is to feed the spectral data into an autoencoder to extract spect...
Embodiment 2
[0050] The scheme in embodiment 1 is verified below in conjunction with concrete experiment, see description below for details:
[0051] 1. Experimental settings
[0052] Datasets and evaluation metrics:
[0053] (1) In this study, the dataset is a total of 60 samples collected from vegetable growing areas in Tianjin. Three types of melons were selected: Elizabeth, Claw, and Emerald. The melons were cut lengthwise and measured for sweetness and firmness in the center.
[0054] Melon sweetness was measured with Brix-90 (refractometer PAL-1). The sweetness value of each sample was measured at 8 points on the melon half: head, tail and side, 4 points closer to the skin and 4 points closer to the pulp. Such as Figure 4 (a) shown. Each sample was tested for hardness values using a texture detector. The firmness of each sample was measured around four locations where the sweetness value was measured. Such as Figure 4 (b) shown.
[0055] For the acquisition of hyperspec...
PUM
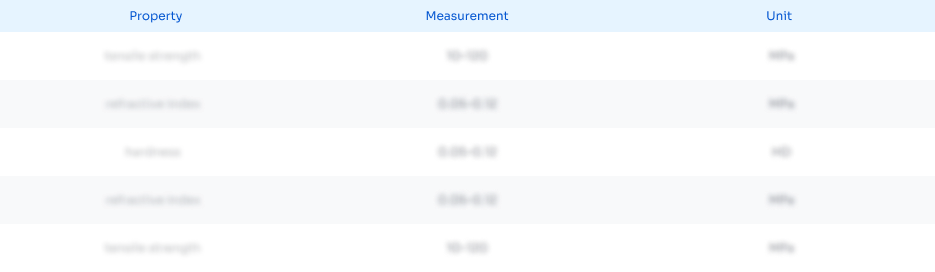
Abstract
Description
Claims
Application Information
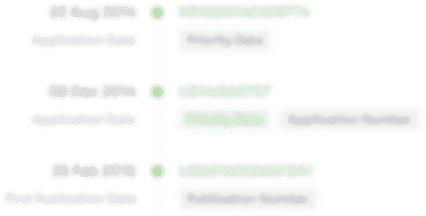
- R&D Engineer
- R&D Manager
- IP Professional
- Industry Leading Data Capabilities
- Powerful AI technology
- Patent DNA Extraction
Browse by: Latest US Patents, China's latest patents, Technical Efficacy Thesaurus, Application Domain, Technology Topic, Popular Technical Reports.
© 2024 PatSnap. All rights reserved.Legal|Privacy policy|Modern Slavery Act Transparency Statement|Sitemap|About US| Contact US: help@patsnap.com