General countermeasure disturbance generation method
A technology of confrontation and perturbation vector, applied in neural learning methods, biological neural network models, instruments, etc., can solve problems such as non-convergence, failure of confrontation samples, generalization ability of confrontation samples, diversity and confrontation strength, etc. Achieve the effect of improving robustness, high misclassification, and strong generalization ability
- Summary
- Abstract
- Description
- Claims
- Application Information
AI Technical Summary
Problems solved by technology
Method used
Image
Examples
Embodiment Construction
[0031] The present invention will now be described in further detail with reference to the drawings. Here, the exemplary embodiments of the present invention and the description thereof are used to explain the present invention, but not as a limitation to the present invention.
[0032] It should be noted that a universal anti-disturbance generation method is characterized by including the following steps:
[0033] Step 1: Obtain the network structure and weight matrix of the target artificial neural network;
[0034] Step 2: Initialize general counter disturbance;
[0035] Step 3: Randomly sample pictures from the training set, superimpose them with the current general counter disturbance and input them into the neural network;
[0036] Step 4: Backpropagation gradients in the current picture label and the label with the highest predicted probability;
[0037] Step 5: Use the prediction vector and two sets of gradients to calculate the update amount against disturbance;
[0038] Step 6:...
PUM
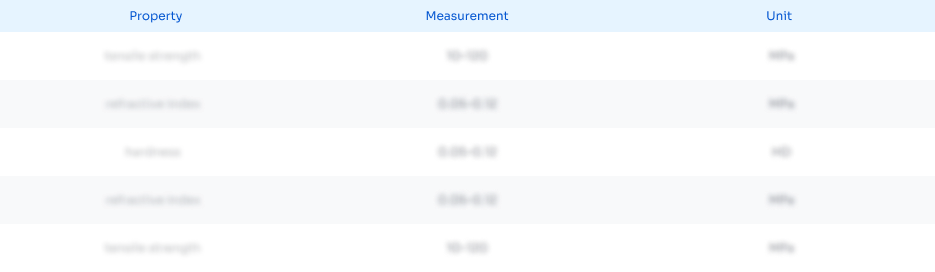
Abstract
Description
Claims
Application Information
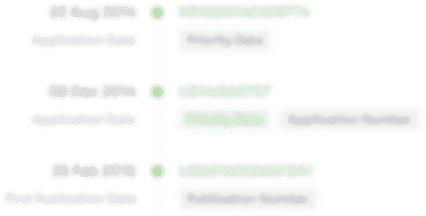
- R&D Engineer
- R&D Manager
- IP Professional
- Industry Leading Data Capabilities
- Powerful AI technology
- Patent DNA Extraction
Browse by: Latest US Patents, China's latest patents, Technical Efficacy Thesaurus, Application Domain, Technology Topic.
© 2024 PatSnap. All rights reserved.Legal|Privacy policy|Modern Slavery Act Transparency Statement|Sitemap