Personality detection method based on multi-modal alignment and multi-vector representation
A detection method and multi-modal technology, applied in the field of data processing, can solve the problems of limited model performance ability, inability to distinguish 5 types of personality well, lack of data volume, etc., to improve performance ability, improve robustness, strengthen The effect of representational ability
- Summary
- Abstract
- Description
- Claims
- Application Information
AI Technical Summary
Problems solved by technology
Method used
Image
Examples
example
[0070] Collect the log files of the three modalities of speech-text-video for the task of personality detection, set up the resampling module, intra-modal representation module, inter-modal alignment representation module, modality fusion module, and prediction module. The resampling module is responsible for sampling the voice and video of the input samples, and obtains a certain number of frames of spectrum and picture input network; the intra-modal representation module is responsible for independently encoding the data of each modality, and obtaining the representation of each modality; The intermodal alignment representation module is responsible for learning the interrelationships between different modalities, and enriching the representation of this modality by using the information aligned with other modalities; the modality fusion module is responsible for fusing the representations learned by the three modalities. One type of personality gets a final vector representa...
PUM
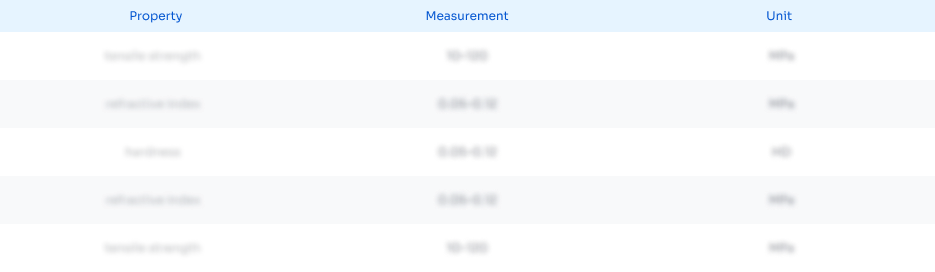
Abstract
Description
Claims
Application Information
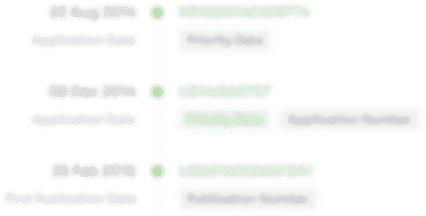
- R&D
- Intellectual Property
- Life Sciences
- Materials
- Tech Scout
- Unparalleled Data Quality
- Higher Quality Content
- 60% Fewer Hallucinations
Browse by: Latest US Patents, China's latest patents, Technical Efficacy Thesaurus, Application Domain, Technology Topic, Popular Technical Reports.
© 2025 PatSnap. All rights reserved.Legal|Privacy policy|Modern Slavery Act Transparency Statement|Sitemap|About US| Contact US: help@patsnap.com