Image restoration model, method and device based on complex task decomposition regularization
A complex task and image technology, applied in the field of image restoration, can solve problems such as difficult to learn image degradation inverse transformation mode, difficult to learn degradation mode, and high difficulty, so as to improve image restoration effect, reduce overfitting problems, and generalize The effect of empowerment
- Summary
- Abstract
- Description
- Claims
- Application Information
AI Technical Summary
Problems solved by technology
Method used
Image
Examples
Embodiment Construction
[0019] The technical solutions in the embodiments of the present application will be described below with reference to the drawings in the embodiments of the present application.
[0020] It should be noted that like numerals and letters denote similar items in the following figures, therefore, once an item is defined in one figure, it does not require further definition and explanation in subsequent figures. Meanwhile, in the description of the present application, the terms "first", "second" and the like are only used to distinguish descriptions, and cannot be understood as indicating or implying relative importance.
[0021] Please see figure 1 , figure 2 , figure 1 A schematic structural diagram of an image restoration model based on complex task decomposition regularization provided by the embodiment of the present application; figure 2 A schematic diagram of a feature extraction and denoising sub-network structure provided by the embodiment of the present applicatio...
PUM
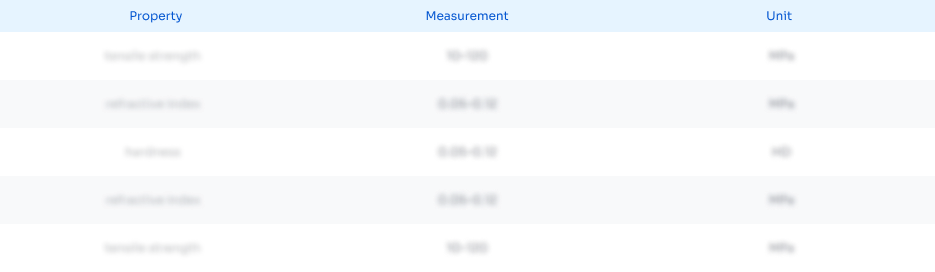
Abstract
Description
Claims
Application Information
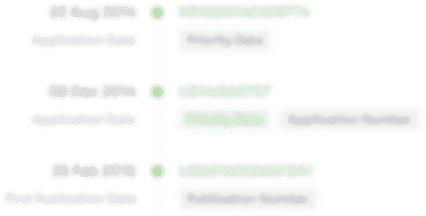
- R&D Engineer
- R&D Manager
- IP Professional
- Industry Leading Data Capabilities
- Powerful AI technology
- Patent DNA Extraction
Browse by: Latest US Patents, China's latest patents, Technical Efficacy Thesaurus, Application Domain, Technology Topic, Popular Technical Reports.
© 2024 PatSnap. All rights reserved.Legal|Privacy policy|Modern Slavery Act Transparency Statement|Sitemap|About US| Contact US: help@patsnap.com