Crop disease image recognition method based on convolutional neural network
A convolutional neural network and image recognition technology, applied in the field of image recognition, can solve real-time, poor accuracy, time-consuming and labor-intensive problems, and achieve the effect of improving early warning of crop diseases
- Summary
- Abstract
- Description
- Claims
- Application Information
AI Technical Summary
Problems solved by technology
Method used
Image
Examples
Embodiment 1
[0025] A method for image recognition of crop diseases based on a convolutional neural network, comprising the steps of:
[0026] S1. Acquisition of crop images at fixed points based on the UAV according to the established cruise route; each crop image will carry supporting POS data, the POS data includes latitude, longitude, elevation, heading angle (Phi), and pitch angle (Omega) and roll angle (Kappa);
[0027] S2. Read the POS data contained in the crop image, and adjust the angle of the image of the diseased area according to the POS data. The angle adjustment includes flipping left and right, flipping up and down, and flipping diagonally, and based on the Faster R-CNN model. Detecting and positioning, generating a diseased area image set; the images in the diseased area image set all carry the hyperlink marks of their corresponding POS data;
[0028] S3. Based on the DSSD_Xception_coco model, the detection and identification of holes, spots, and pest tracks in the image ...
Embodiment 2
[0032] A method for image recognition of crop diseases based on a convolutional neural network, comprising the steps of:
[0033] S1. Acquisition of crop images at fixed points based on the UAV according to the established cruise route; each crop image will carry supporting POS data, the POS data includes latitude, longitude, elevation, heading angle (Phi), and pitch angle (Omega) and roll angle (Kappa);
[0034] S2. Read the POS data contained in the crop image, and adjust the angle of the image of the diseased area according to the POS data. The angle adjustment includes flipping left and right, flipping up and down, and flipping diagonally, and based on the Faster R-CNN model. Detecting and positioning, generating a diseased area image set; the images in the diseased area image set all carry the hyperlink marks of their corresponding POS data;
[0035] S3. Based on the DSSD_Xception_coco model, the detection and identification of holes, spots, and pest tracks in the image ...
Embodiment 3
[0040] A method for image recognition of crop diseases based on a convolutional neural network, comprising the steps of:
[0041] S1. Acquisition of crop images at fixed points based on the UAV according to the established cruise route; each crop image will carry supporting POS data, the POS data includes latitude, longitude, elevation, heading angle (Phi), and pitch angle (Omega) and roll angle (Kappa);
[0042] S2. Read the POS data contained in the crop image, and adjust the angle of the image of the diseased area according to the POS data. The angle adjustment includes flipping left and right, flipping up and down, and flipping diagonally, and based on the Faster R-CNN model. Detecting and positioning, generating a diseased area image set; the images in the diseased area image set all carry the hyperlink marks of their corresponding POS data;
[0043] S3. Based on the DSSD_Xception_coco model, the detection and identification of holes, spots, and pest tracks in the image ...
PUM
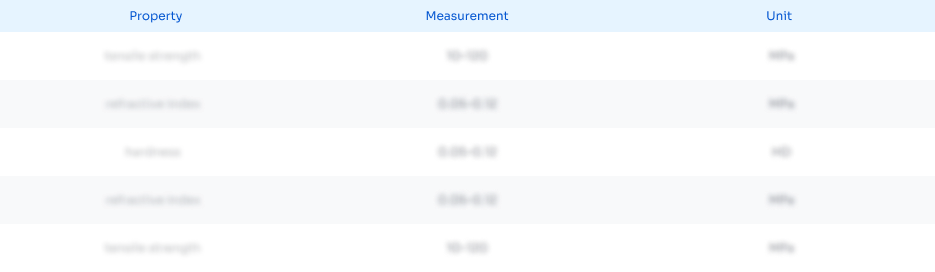
Abstract
Description
Claims
Application Information
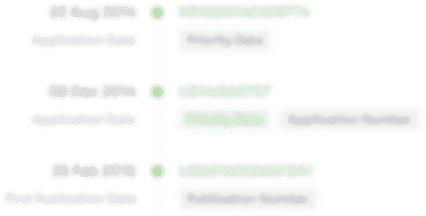
- R&D
- Intellectual Property
- Life Sciences
- Materials
- Tech Scout
- Unparalleled Data Quality
- Higher Quality Content
- 60% Fewer Hallucinations
Browse by: Latest US Patents, China's latest patents, Technical Efficacy Thesaurus, Application Domain, Technology Topic, Popular Technical Reports.
© 2025 PatSnap. All rights reserved.Legal|Privacy policy|Modern Slavery Act Transparency Statement|Sitemap|About US| Contact US: help@patsnap.com