Lithium battery surface defect detection method based on depth field adaptation
A defect detection, lithium battery technology, applied in neural learning methods, optical testing flaws/defects, measuring devices, etc., can solve problems such as unsatisfactory recognition rate, achieve reduced processing power, low computing cost, and strong generalization ability Effect
- Summary
- Abstract
- Description
- Claims
- Application Information
AI Technical Summary
Problems solved by technology
Method used
Image
Examples
Embodiment Construction
[0053] The following will clearly and completely describe the technical solutions in the embodiments of the present invention with reference to the drawings in the embodiments of the present invention. Based on the embodiments of the present invention, all other embodiments obtained by persons of ordinary skill in the art without making creative efforts belong to the protection scope of the present invention.
[0054] Such as figure 1 As shown, it is a lithium battery surface defect detection model based on deep domain adaptation involved in the present invention, and the model includes three sub-modules: feature extractor, classifier and domain discriminator. This model is a new domain adaptation model that combines the minimization of statistics and adversarial discriminative methods to achieve distribution alignment.
[0055] The feature extractor is a sub-module built using a convolutional neural network to extract effective features of input samples. The feature extract...
PUM
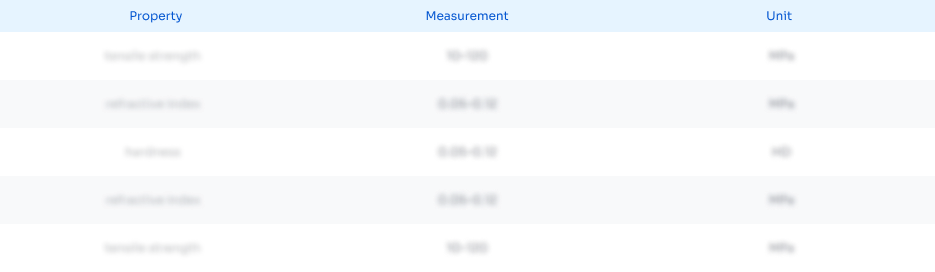
Abstract
Description
Claims
Application Information
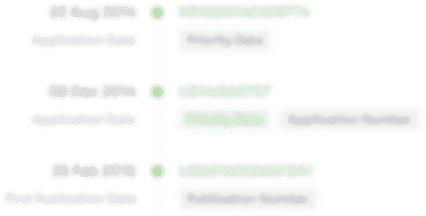
- R&D Engineer
- R&D Manager
- IP Professional
- Industry Leading Data Capabilities
- Powerful AI technology
- Patent DNA Extraction
Browse by: Latest US Patents, China's latest patents, Technical Efficacy Thesaurus, Application Domain, Technology Topic, Popular Technical Reports.
© 2024 PatSnap. All rights reserved.Legal|Privacy policy|Modern Slavery Act Transparency Statement|Sitemap|About US| Contact US: help@patsnap.com