Image segmentation method, device and equipment and storage medium
An image segmentation and superpixel segmentation technology, applied in the field of computer vision, can solve the problems of complete object recognition, lack of full consideration of relevance, and inability to accurately locate the edge of the picture, so as to improve the prediction accuracy and increase the calculation cost.
- Summary
- Abstract
- Description
- Claims
- Application Information
AI Technical Summary
Problems solved by technology
Method used
Image
Examples
Embodiment
[0027] When the image segmentation model based on full convolutional neural network training is used for image semantic segmentation, the prediction accuracy of the image segmentation model is not high due to inaccurate image edge positioning and lack of spatial consistency. The inaccurate positioning of the edges of the above pictures and the lack of spatial consistency are ultimately due to the fact that the image segmentation model based on the training of the fully convolutional neural network cannot recognize the underlying features of the picture such as color, brightness, texture, and gradient. Based on the above, it can be considered how to make the image segmentation model generated based on full convolutional neural network training can accurately identify the underlying features of the picture, thereby improving the prediction accuracy of the image segmentation model.
[0028] In traditional methods, image semantic segmentation algorithms based on superpixel segmenta...
PUM
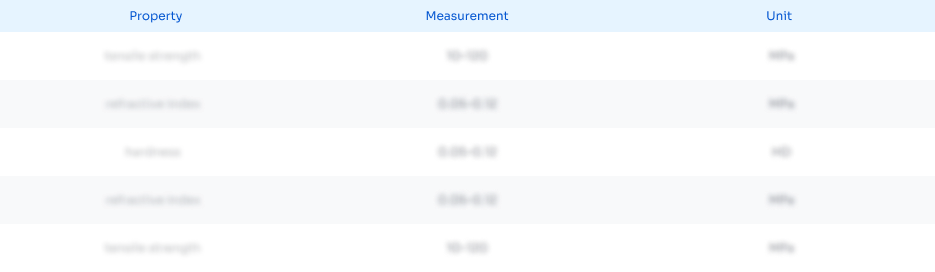
Abstract
Description
Claims
Application Information
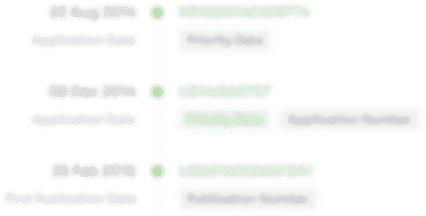
- R&D Engineer
- R&D Manager
- IP Professional
- Industry Leading Data Capabilities
- Powerful AI technology
- Patent DNA Extraction
Browse by: Latest US Patents, China's latest patents, Technical Efficacy Thesaurus, Application Domain, Technology Topic, Popular Technical Reports.
© 2024 PatSnap. All rights reserved.Legal|Privacy policy|Modern Slavery Act Transparency Statement|Sitemap|About US| Contact US: help@patsnap.com