Vehicle type identification method based on machine vision and deep learning
A deep learning and vehicle type technology, applied in neural learning methods, character and pattern recognition, instruments, etc., can solve problems such as high error rate, serious occlusion, overlapping vehicles, etc., improve accuracy and precision, and reduce recognition and detection errors , Good detection effect
- Summary
- Abstract
- Description
- Claims
- Application Information
AI Technical Summary
Problems solved by technology
Method used
Image
Examples
Embodiment Construction
[0049] The following is a detailed description in conjunction with the machine vision-based traffic road vehicle type detection system of the present invention and the accompanying drawings, so as to clearly and completely describe the technical solutions in the implementation examples of the present invention.
[0050] A kind of residual yarn detection method based on machine vision is proposed in the example of the present invention, as figure 1As shown, in the specific scheme, it can be divided into three major steps: image acquisition and preprocessing, yolov3 preliminary detection target and classifier re-identification. First, it is necessary to use mobile platforms and industrial cameras to capture images from traffic road scenes, and perform preprocessing such as Gaussian filtering on the collected images. Then send the preprocessed image into the yolov3 target detector and get the preliminary detection frame and confidence, and then determine whether to send it to mul...
PUM
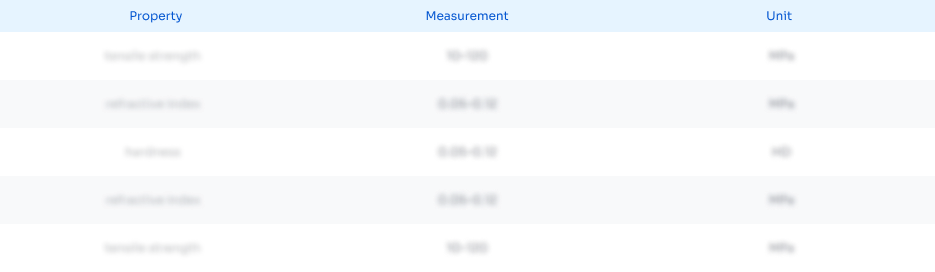
Abstract
Description
Claims
Application Information
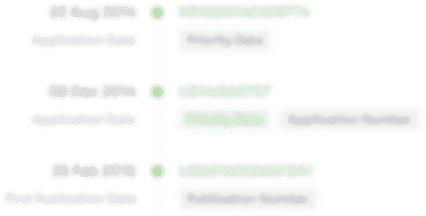
- R&D
- Intellectual Property
- Life Sciences
- Materials
- Tech Scout
- Unparalleled Data Quality
- Higher Quality Content
- 60% Fewer Hallucinations
Browse by: Latest US Patents, China's latest patents, Technical Efficacy Thesaurus, Application Domain, Technology Topic, Popular Technical Reports.
© 2025 PatSnap. All rights reserved.Legal|Privacy policy|Modern Slavery Act Transparency Statement|Sitemap|About US| Contact US: help@patsnap.com