Zero-sample image classification method based on regression variation auto-encoder
A technology of self-encoder and sample image, which is applied in neural learning methods, instruments, computer components, etc.
- Summary
- Abstract
- Description
- Claims
- Application Information
AI Technical Summary
Problems solved by technology
Method used
Image
Examples
Embodiment
[0074] The first step, data preprocessing:
[0075] When solving the generalized zero-sample image classification problem, a part of the known class data set in the database is used as a training set, and the other part and an unknown class data set are used as a test set, and the data used include known class image features v seen Data and known class semantic features c seen data, and unknown class image features v unseen Data and Unknown Class Semantic Features c unseen Data, this embodiment is tested on 4 data sets, namely AWA1, AWA2, CUB and SUN, all data are from the document "Zero-Shot Learning-A Comprehensive Evaluation of the Good, the Bad and the Ugly" published Available data, all image features come from the 2048-dimensional final pooling layer of the well-known residual network ResNet-101, and use manual labeling attribute information as semantic features to complete data preprocessing;
[0076] In the second step, train the aligned cross reconstruction variati...
PUM
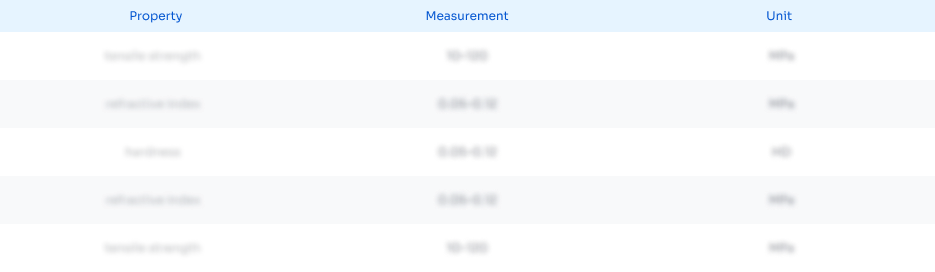
Abstract
Description
Claims
Application Information
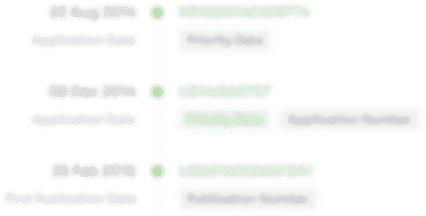
- R&D
- Intellectual Property
- Life Sciences
- Materials
- Tech Scout
- Unparalleled Data Quality
- Higher Quality Content
- 60% Fewer Hallucinations
Browse by: Latest US Patents, China's latest patents, Technical Efficacy Thesaurus, Application Domain, Technology Topic, Popular Technical Reports.
© 2025 PatSnap. All rights reserved.Legal|Privacy policy|Modern Slavery Act Transparency Statement|Sitemap|About US| Contact US: help@patsnap.com