Cross-modal generalized zero sample retrieval method based on dual learning generative adversarial network
A cross-modal, network model technology, applied in the field of cross-modal retrieval, can solve the problems of information loss, semantic information loss, inability to encode, etc., and achieve the effect of reducing cumbersomeness and expensive cost and improving retrieval effect.
- Summary
- Abstract
- Description
- Claims
- Application Information
AI Technical Summary
Problems solved by technology
Method used
Image
Examples
Embodiment Construction
[0036] In order to make the purpose, technical solution and advantages of the present invention more clear, the specific implementation manners of the present invention will be further described in detail below in conjunction with the accompanying drawings, so that those skilled in the art can better understand the present invention. It should be noted that the described embodiments are some, not all, embodiments of the present invention, and are not intended to limit the scope of the claimed invention. All other embodiments obtained by persons of ordinary skill in the art without creative efforts fall within the protection scope of the present invention.
[0037] Such as figure 1 As shown, in the present invention, the cross-modal generalization zero-shot retrieval method based on generative confrontation network includes the following steps:
[0038] Step 1: Select the training dataset. In this example, two large-scale datasets Sketchy Ext. and TU-Berlin Ext. were selected...
PUM
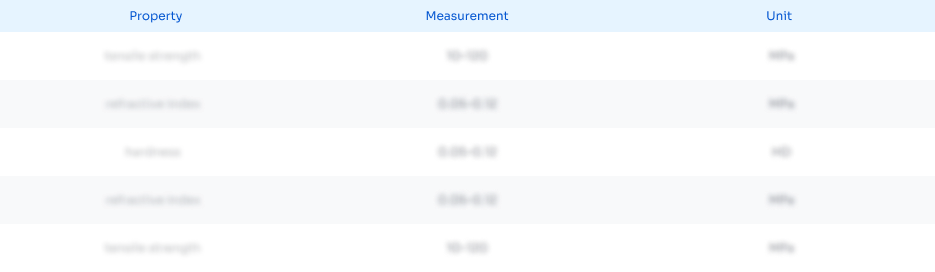
Abstract
Description
Claims
Application Information
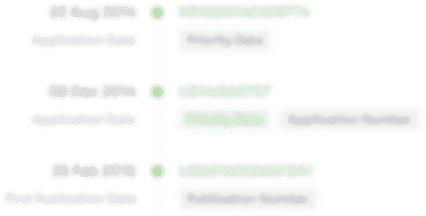
- R&D
- Intellectual Property
- Life Sciences
- Materials
- Tech Scout
- Unparalleled Data Quality
- Higher Quality Content
- 60% Fewer Hallucinations
Browse by: Latest US Patents, China's latest patents, Technical Efficacy Thesaurus, Application Domain, Technology Topic, Popular Technical Reports.
© 2025 PatSnap. All rights reserved.Legal|Privacy policy|Modern Slavery Act Transparency Statement|Sitemap|About US| Contact US: help@patsnap.com