Fault diagnosis method based on improved convolutional neural network
A convolutional neural network and fault diagnosis model technology, applied to biological neural network models, neural architectures, instruments, etc., can solve the problems of gradient disappearance, large amount of calculation, weak model generalization ability, etc., to reduce size and enhance generalization The effect of the ability
- Summary
- Abstract
- Description
- Claims
- Application Information
AI Technical Summary
Problems solved by technology
Method used
Image
Examples
Embodiment Construction
[0012] The specific embodiments of the present invention will be further described below in conjunction with the accompanying drawings.
[0013] The application provides a fault diagnosis method based on an improved convolutional neural network, the method comprising the steps of:
[0014] Step S1, obtain a sample data set, perform data preprocessing on the sample data set to obtain a training set and a test set, wherein the sample data set includes time series data of each state type, and the state types of this application include normal state and q-type fault state, If q is a positive integer, the obtained sample data set can be expressed as {time series data of normal state, time series data of fault state 1, time series data of fault state 2...time series data of fault state q}, each A set of time series data consists of several data points.
[0015] The method for preparing a training set and a test set based on a sample data set includes the following steps S1a-S1d:
...
PUM
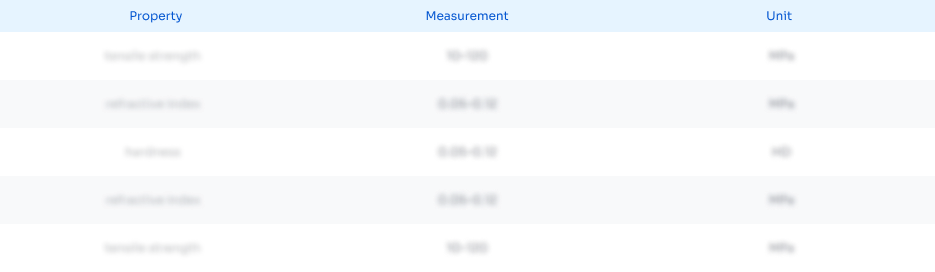
Abstract
Description
Claims
Application Information
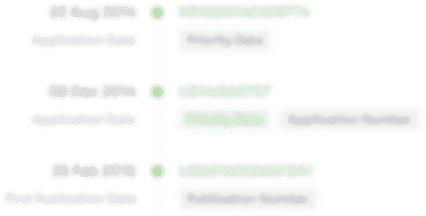
- R&D Engineer
- R&D Manager
- IP Professional
- Industry Leading Data Capabilities
- Powerful AI technology
- Patent DNA Extraction
Browse by: Latest US Patents, China's latest patents, Technical Efficacy Thesaurus, Application Domain, Technology Topic, Popular Technical Reports.
© 2024 PatSnap. All rights reserved.Legal|Privacy policy|Modern Slavery Act Transparency Statement|Sitemap|About US| Contact US: help@patsnap.com