An Efficient Value Function Iterative Reinforcement Learning Method for Shared Recurrent Neural Networks
A recurrent neural network and reinforcement learning technology, applied in the field of efficient value function iterative reinforcement learning, can solve the problems of long interaction time and high sampling cost
- Summary
- Abstract
- Description
- Claims
- Application Information
AI Technical Summary
Problems solved by technology
Method used
Image
Examples
Embodiment Construction
[0058] Embodiments of the present invention are described in detail below, examples of which are shown in the drawings, wherein the same or similar reference numerals designate the same or similar elements or elements having the same or similar functions throughout. The embodiments described below by referring to the figures are exemplary and are intended to explain the present invention and should not be construed as limiting the present invention.
[0059] The following describes an iterative reinforcement learning method for high-efficiency value function sharing a cyclic neural network according to an embodiment of the present invention with reference to the accompanying drawings.
[0060] First of all, the high-efficiency value function iterative reinforcement learning method of the shared cyclic neural network proposed by the present invention includes two modules: a Critic module and an Actor module. In the Critic module, the problem of overestimation of the value funct...
PUM
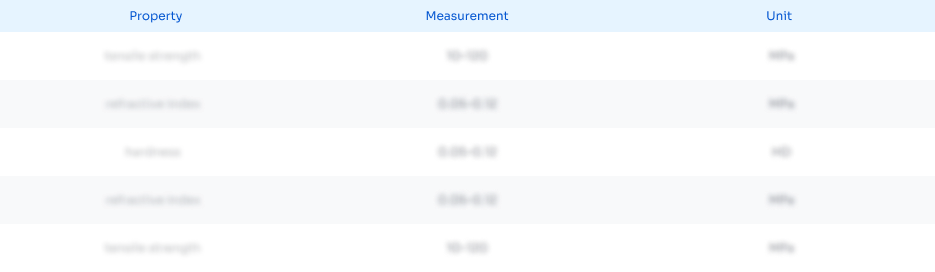
Abstract
Description
Claims
Application Information
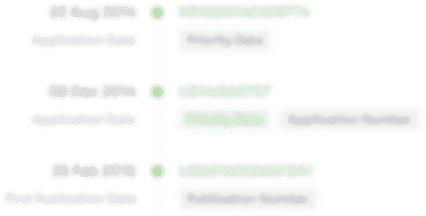
- R&D Engineer
- R&D Manager
- IP Professional
- Industry Leading Data Capabilities
- Powerful AI technology
- Patent DNA Extraction
Browse by: Latest US Patents, China's latest patents, Technical Efficacy Thesaurus, Application Domain, Technology Topic, Popular Technical Reports.
© 2024 PatSnap. All rights reserved.Legal|Privacy policy|Modern Slavery Act Transparency Statement|Sitemap|About US| Contact US: help@patsnap.com