Multi-agent cooperation information processing method and system, storage medium and intelligent terminal
An information processing method and multi-agent technology, applied in neural learning methods, biological neural network models, instruments, etc., can solve problems such as difficulty in training, increasing algorithm convergence time, and slow learning.
- Summary
- Abstract
- Description
- Claims
- Application Information
AI Technical Summary
Problems solved by technology
Method used
Image
Examples
Embodiment 1
[0210] Embodiment 1, urban planning. When there are a large number of vehicles in the city, in order to reduce the overall urban traffic congestion time, deep multi-agent reinforcement learning is used to recommend the optimal travel route for each vehicle to ensure smooth traffic. Optimize bus routes and optimize traffic light control. Such as Figure 13 shown.
[0211] Step 1: Construct the road network of Mianyang City.
[0212] The second step: analyze the OD of the driving trajectory. The driving trajectory is mapped to the road network.
[0213] The third step: analysis system of time and space law of motor vehicle travel in Mianyang City based on bayonet data.
[0214] Step 4: Take the OD heat map of the driving trajectory, such as Figure 14 shown.
[0215] Step 5: Use the GAED-MADDPG algorithm to find the optimal traffic organization scheme, such as Figure 15 shown.
[0216] It should be noted that the embodiments of the present invention can be realized by ...
PUM
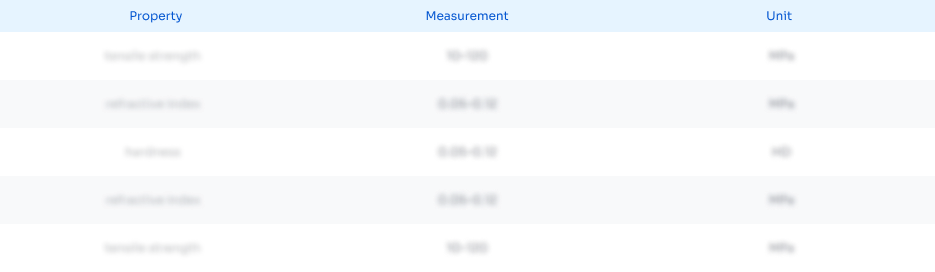
Abstract
Description
Claims
Application Information
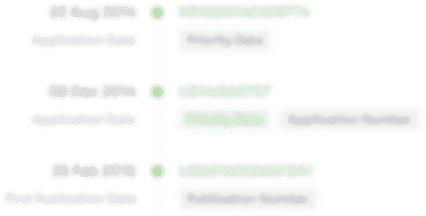
- R&D Engineer
- R&D Manager
- IP Professional
- Industry Leading Data Capabilities
- Powerful AI technology
- Patent DNA Extraction
Browse by: Latest US Patents, China's latest patents, Technical Efficacy Thesaurus, Application Domain, Technology Topic.
© 2024 PatSnap. All rights reserved.Legal|Privacy policy|Modern Slavery Act Transparency Statement|Sitemap