Wireless sensor network topology optimization method based on asynchronous deep reinforcement learning
A wireless sensor and reinforcement learning technology, applied in network topology, neural learning methods, network planning, etc., can solve the problems of data optimization results oscillation, dependence on computing resources, etc., to speed up the convergence time, improve the ability to resist attacks, improve communication effect of ability
- Summary
- Abstract
- Description
- Claims
- Application Information
AI Technical Summary
Problems solved by technology
Method used
Image
Examples
Embodiment Construction
[0018] The specific method, structure, features, and functions of the wireless sensor network topology optimization method designed according to the present invention are described in detail below in conjunction with the accompanying drawings.
[0019] Step 1: Use the rules of the scale-free network model to generate the initialized wireless sensor network topology X. The network topology nodes are randomly deployed, and the nodes newly added to the wireless sensor network are connected according to the edge density parameter M. Newly added nodes are connected to existing nodes with high probability first, ensuring that the wireless sensor network can describe the network topology characteristics of the real world to the greatest extent, and at the same time, the geographic location P of the node is fixed. Each node has the same attributes.
[0020] Among them, setting the edge density parameter to M=2 means that the number of edges in the wireless sensor network is twice the numb...
PUM
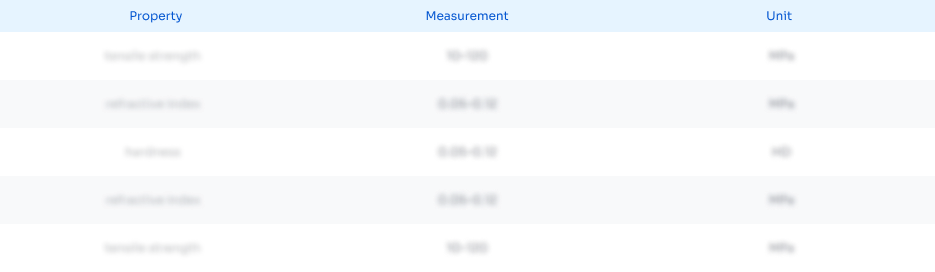
Abstract
Description
Claims
Application Information
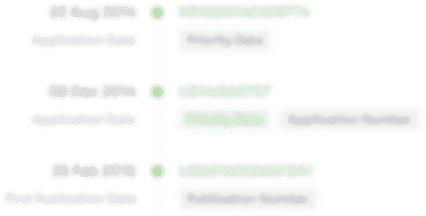
- R&D Engineer
- R&D Manager
- IP Professional
- Industry Leading Data Capabilities
- Powerful AI technology
- Patent DNA Extraction
Browse by: Latest US Patents, China's latest patents, Technical Efficacy Thesaurus, Application Domain, Technology Topic, Popular Technical Reports.
© 2024 PatSnap. All rights reserved.Legal|Privacy policy|Modern Slavery Act Transparency Statement|Sitemap|About US| Contact US: help@patsnap.com