Complex chemical process fault diagnosis method based on deep learning multi-model fusion
A chemical process and fault diagnosis technology, applied in program control, instrumentation, electrical testing/monitoring, etc., can solve the problem of huge amount of data, low precision of chemical process fault diagnosis, and difficulty in diagnosis accuracy or diagnosis time to meet the requirements of fault diagnosis, etc. problem, to achieve the effect of overcoming the large amount of calculation
- Summary
- Abstract
- Description
- Claims
- Application Information
AI Technical Summary
Problems solved by technology
Method used
Image
Examples
Embodiment
[0043] The TE process is a complex chemical simulation process model proposed by Eastman Chemical Company of the United States, which contains 41 measured variables and 12 control variables, but the 12th control variable, the stirring speed, is constant and is not considered. All process measurements The values all contain Gaussian noise, preset 21 faults, faults 1 to 7 are related to step changes in process variables, faults 8 to 12 are related to variability of some process variables, and fault 13 reflects slow drift in dynamics, Faults 14, 15, and 21 are related to valve sticking.
[0044] The specific operation of applying the method of the present invention to the above-mentioned TE process simulation object is as follows.
[0045] Step 1. Preprocess the experimental data set
[0046] 1-1. Each fault type and normal state contains 1280 samples. After labeling the experimental data set, randomly scramble it, observe the correlation between the sample feature points and ...
PUM
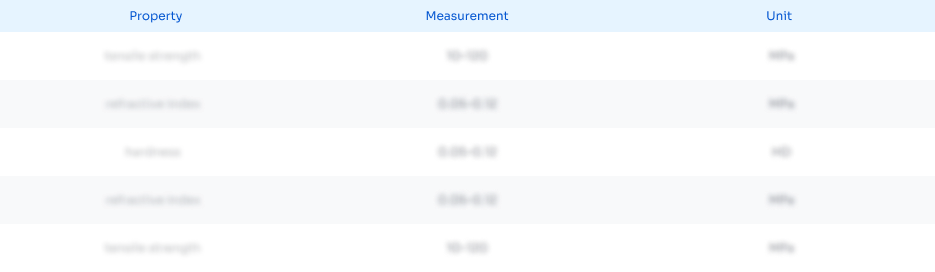
Abstract
Description
Claims
Application Information
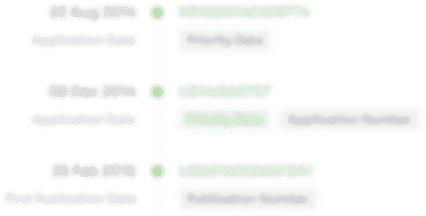
- R&D Engineer
- R&D Manager
- IP Professional
- Industry Leading Data Capabilities
- Powerful AI technology
- Patent DNA Extraction
Browse by: Latest US Patents, China's latest patents, Technical Efficacy Thesaurus, Application Domain, Technology Topic.
© 2024 PatSnap. All rights reserved.Legal|Privacy policy|Modern Slavery Act Transparency Statement|Sitemap