Chinese named entity identification data enhancement algorithm based on sequence generative adversarial network
A technology for named entity recognition and sequence generation, applied in the Internet field, can solve problems such as spending a lot of manpower and time, lack of a large amount of labeled data, and unsolved problems.
- Summary
- Abstract
- Description
- Claims
- Application Information
AI Technical Summary
Problems solved by technology
Method used
Image
Examples
Embodiment 1
[0061] refer to figure 1 , 2 As shown, the present invention provides a method for applying a data enhancement algorithm based on a sequence generation confrontation network to a named entity recognition task. Specifically, during training, the method includes:
[0062] Step 1: Process the sentences in the corpus, divide each sentence into entity and non-entity parts according to the entity label information of the sentence, and add the entity and non-entity parts to the dictionary at the same time. Suppose a text sequence {c 1 ,c 2 ,c 3 ,c 4 ,c 5 ,c 6} label is {O,O,B-PER,I-PER,O,O}, you can put c 1 c 2 ,c 5 c 6 Classified as non-substantial parts, c 3 c 4 into entity parts, and then add them and their corresponding labels to the dictionary.
[0063] Step 2: According to the dictionary formed by entities and non-entities, the entities and non-entities in each sentence are mapped to corresponding indexes in the dictionary to form an index sequence.
[0064] Step ...
PUM
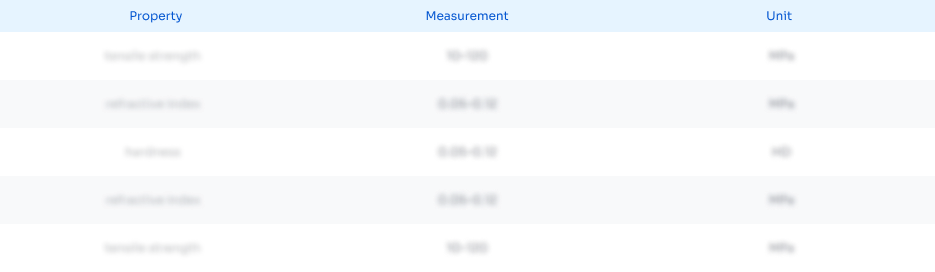
Abstract
Description
Claims
Application Information
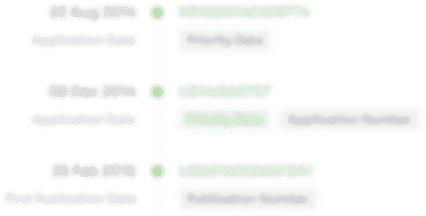
- R&D
- Intellectual Property
- Life Sciences
- Materials
- Tech Scout
- Unparalleled Data Quality
- Higher Quality Content
- 60% Fewer Hallucinations
Browse by: Latest US Patents, China's latest patents, Technical Efficacy Thesaurus, Application Domain, Technology Topic, Popular Technical Reports.
© 2025 PatSnap. All rights reserved.Legal|Privacy policy|Modern Slavery Act Transparency Statement|Sitemap|About US| Contact US: help@patsnap.com