Multi-task learning method and system based on feature and sample adversarial symbiosis
A multi-task learning and sample technology, applied in the multi-task learning method and system field based on feature and sample confrontation symbiosis, can solve problems such as domain distribution differences, improve generalization performance, solve domain distribution differences and small sample problems Effect
- Summary
- Abstract
- Description
- Claims
- Application Information
AI Technical Summary
Problems solved by technology
Method used
Image
Examples
Embodiment Construction
[0024] In order to make the purpose, technical solutions and advantages of the embodiments of the present invention clearer, the technical solutions in the embodiments of the present invention will be clearly and completely described below in conjunction with the drawings in the embodiments of the present invention. Obviously, the described embodiments It is a part of embodiments of the present invention, but not all embodiments. Based on the embodiments of the present invention, all other embodiments obtained by persons of ordinary skill in the art without creative efforts fall within the protection scope of the present invention.
[0025] Such as figure 1 As shown, the present invention provides a multi-task learning method based on feature and sample confrontation symbiosis, comprising the following steps:
[0026] S1. Randomly extract samples of tasks to generate common implicit features that have nothing to do with the domain;
[0027] S2. Generate highly simulated samp...
PUM
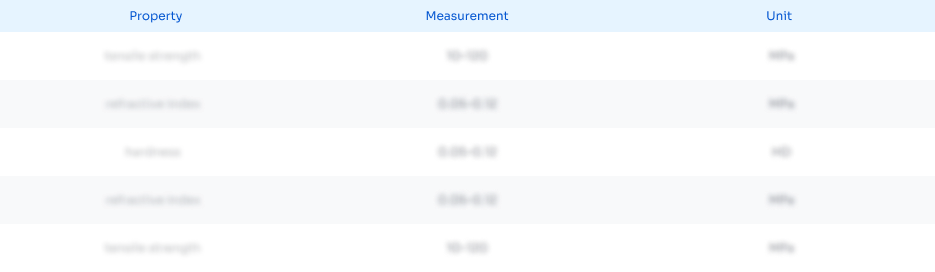
Abstract
Description
Claims
Application Information
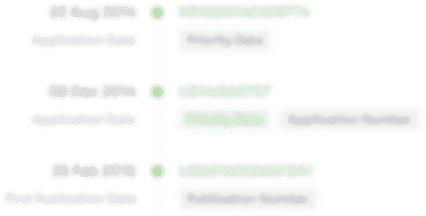
- R&D
- Intellectual Property
- Life Sciences
- Materials
- Tech Scout
- Unparalleled Data Quality
- Higher Quality Content
- 60% Fewer Hallucinations
Browse by: Latest US Patents, China's latest patents, Technical Efficacy Thesaurus, Application Domain, Technology Topic, Popular Technical Reports.
© 2025 PatSnap. All rights reserved.Legal|Privacy policy|Modern Slavery Act Transparency Statement|Sitemap|About US| Contact US: help@patsnap.com