An adaptive soft sensing method and system based on vine copula quantile regression
A quantile regression, soft measurement technology, applied in adaptive control, general control system, control/regulation system, etc., can solve problems such as large model error, improve practicability, good regression prediction ability, and avoid information loss Effect
- Summary
- Abstract
- Description
- Claims
- Application Information
AI Technical Summary
Problems solved by technology
Method used
Image
Examples
Embodiment 1
[0134] The invention discloses an adaptive soft measurement method based on vine copula quantile regression, and the specific steps are as follows:
[0135] [Step S1]: According to the actual industrial production situation and expert knowledge, obtain data under normal operating conditions, and select appropriate auxiliary variables for the soft sensing model;
[0136] [Step S2]: Normalize the training data to obtain data that can be used for copula modeling. To normalize the data, see equation (15)
[0137]
[0138] where X i is the variable before transformation, X i ' is the zero-mean standardized variable, mean(X i ) is the variable X i The mean of , sd(X i ) is the variable X i The standard deviation of , m is the dimension of the vector X.
[0139] [Step S3]: Use D-vine copula to establish a quantile regression model.
[0140] Step 3.1: Formula (16) constructs the analytical model of D-vine copula:
[0141]
[0142] (F(X j |X j+1 ,…,X j+i-1 ),F(X j+i ...
Embodiment 2
[0184] The following examples will help to understand the present invention, but do not limit the content of the present invention. see figure 2 , this embodiment realizes the prediction of the numerical instance, and the data of this embodiment is generated according to formula (25).
[0185] x i ~N(0,1)i=1,2
[0186]
[0187] x 4 =sin(x 2 +1)+x 2 x 3
[0188]
[0189] And the error e~N(0,0.01) is added to each dimension data. The prediction target is y. A total of 500 sets of data are selected, 400 sets are used for training the copula model, and 100 sets are used for testing.
[0190] (1) According to the prior information, four auxiliary variables are selected as x in formula (24). 1 ,x 2 ,x 3 ,x 4 , the key variable is y. (2) Data preprocessing: normalize the zero mean of the training samples to obtain the transformed data as [V, U 1 , U 2 , U 3 , U 4 ]. where V represents the normalized key variable, U 1 , U 2 , U 3 , U 4 stands for auxiliary...
Embodiment 3
[0203] see Figure 4 , This example realizes the prediction (PER) of the ethylene cracking degree in the ethylene cracking process. The data of this example comes from the SRT-III model ethylene cracking furnace. 500 sets of data under normal conditions were selected, 400 sets were used to train the copula model, and 100 sets were used for testing.
[0204] (1) According to the prior information, four auxiliary variables are selected: the average outlet temperature of the cracking furnace x 1 , the density of the pyrolysis feedstock x 2 , total feed x 3 and steam hydrocarbon ratio x 4 . The key variable y is the cracking depth indicator PER.
[0205] (2) Data preprocessing: normalize the zero mean of the training samples to obtain the transformed data [V, U 1 , U 2 , U 3 , U 4 ].
[0206] (3) Using the training samples [V, U 1 , U 2 , U 3 , U 4 ] Build the D-vine copula.
[0207] (4) Establish a quantile regression model, calculate the inverse function value of ...
PUM
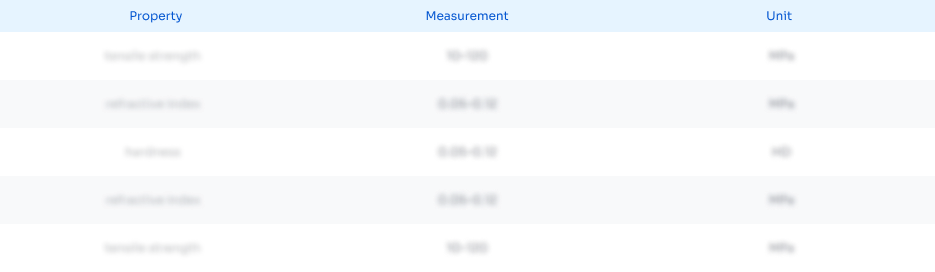
Abstract
Description
Claims
Application Information
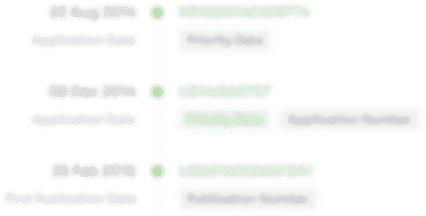
- R&D
- Intellectual Property
- Life Sciences
- Materials
- Tech Scout
- Unparalleled Data Quality
- Higher Quality Content
- 60% Fewer Hallucinations
Browse by: Latest US Patents, China's latest patents, Technical Efficacy Thesaurus, Application Domain, Technology Topic, Popular Technical Reports.
© 2025 PatSnap. All rights reserved.Legal|Privacy policy|Modern Slavery Act Transparency Statement|Sitemap|About US| Contact US: help@patsnap.com