Sleep staging method based on deep learning
A technology of sleep staging and deep learning, which is applied in the field of sleep staging based on deep learning, can solve the problems of low average recognition rate of sleep categories, and achieve the effect of avoiding low recognition rate, increasing receptive field, and retaining information
- Summary
- Abstract
- Description
- Claims
- Application Information
AI Technical Summary
Problems solved by technology
Method used
Image
Examples
Embodiment Construction
[0039] The details of the present invention will be further described below in conjunction with the accompanying drawings and examples.
[0040] The present invention proposes a sleep staging method based on deep learning, such as figure 1 As shown, it specifically includes the following steps:
[0041] Step 1: Obtain a single-channel EEG signal to construct a data set, and oversample the EEG signal in the data set, and divide the oversampled EEG signal data set into a training set and a verification set; specifically include:
[0042] Obtain the sleep staging data set Sleep-EDF on the Internet. According to the standards of the American Academy of Sleep Medicine, the labels of sleep periods are divided into 5 categories, namely awakening period, light sleep stage I, light sleep stage II, deep sleep period, Rapid eye movement period; because part of the record file is up to 20 hours, this embodiment pays attention to the sleep situation at night, so the data interception is c...
PUM
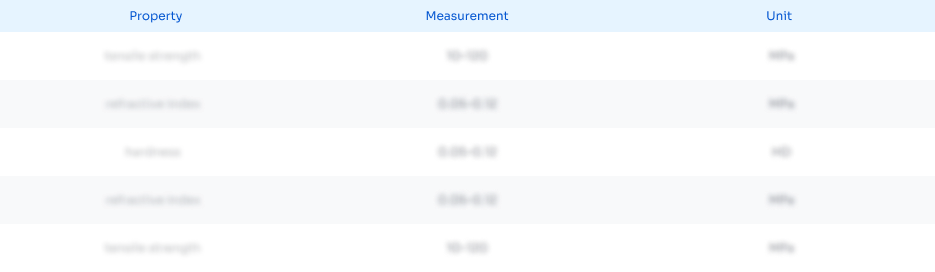
Abstract
Description
Claims
Application Information
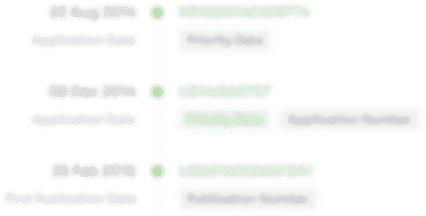
- R&D Engineer
- R&D Manager
- IP Professional
- Industry Leading Data Capabilities
- Powerful AI technology
- Patent DNA Extraction
Browse by: Latest US Patents, China's latest patents, Technical Efficacy Thesaurus, Application Domain, Technology Topic, Popular Technical Reports.
© 2024 PatSnap. All rights reserved.Legal|Privacy policy|Modern Slavery Act Transparency Statement|Sitemap|About US| Contact US: help@patsnap.com