Depression patient identification system and identification method thereof
An identification system and identification method technology, applied in the identification system of depression patients and their identification field, can solve problems such as difficulties in early screening of depression, and achieve the effect of promoting prevention and improving accuracy
- Summary
- Abstract
- Description
- Claims
- Application Information
AI Technical Summary
Problems solved by technology
Method used
Image
Examples
Embodiment 1
[0039] Such as Figure 1-3 As shown, the present invention is a depression patient identification system and its identification method, which specifically includes the following implementation steps:
[0040] S1: Collect and organize the data, construct the original data set, the data is the EWAS analysis result of the DNA methylation of the candidate gene;
[0041] S2: Data preprocessing is performed on the original data set, and the input data set is obtained after discarding missing values;
[0042] S3: Randomly divide the input data set into training data set and test data set according to the ratio of 0.8:0.2;
[0043] S4: Create a deep learning model to identify patients with depression and healthy people, and use the training data set to train the constructed deep learning model;
[0044] S5: Use the test set to evaluate the performance of the trained deep learning model, and continuously optimize the model during the verification evaluation process to obtain the opti...
Embodiment 2
[0058] Such as Figure 4 As shown, based on the method in Example 1, a total of 391 samples of 291 depression patients and 100 healthy controls who met the criteria were tested, and the results were calculated for comparison.
[0059] 1. Perform methylation sequencing of candidate genes on 391 samples that meet the criteria for inclusion. The candidate genes are: HTR1A, HTR1B, S100A10 and BDNF. Quality control and analysis of the sequencing results will result in EWAS analysis of DNA methylation The result is used as the original data set, in which the number of sequencing sites is 449;
[0060] 2. Raw data set for data preprocessing. Considering the reasons for the deletion of some sequencing sites, the filling cannot reflect the real sequencing results, so the missing values are directly discarded. After missing values are processed, the number of corresponding sequencing sites is 406, and the number of samples is 333;
[0061] 3. Divide the input data obtained after ...
Embodiment 3
[0066] Such as Figure 5 As shown, a system for identifying patients with depression based on a deep learning model is proposed, including:
[0067] A: data preprocessing unit, which can preprocess the EWAS analysis results of candidate gene DNA methylation sequencing;
[0068] B: Model creation unit, which can create a deep learning model to identify patients with depression and healthy people
[0069] C: a model optimization unit, which can optimize the deep learning model;
[0070] D: data identification unit, which can effectively identify the methylation sequencing data to be distinguished based on the optimized deep learning model.
PUM
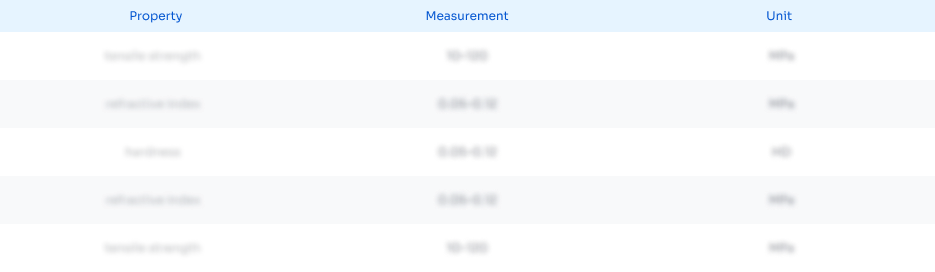
Abstract
Description
Claims
Application Information
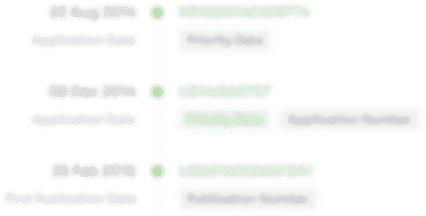
- R&D Engineer
- R&D Manager
- IP Professional
- Industry Leading Data Capabilities
- Powerful AI technology
- Patent DNA Extraction
Browse by: Latest US Patents, China's latest patents, Technical Efficacy Thesaurus, Application Domain, Technology Topic, Popular Technical Reports.
© 2024 PatSnap. All rights reserved.Legal|Privacy policy|Modern Slavery Act Transparency Statement|Sitemap|About US| Contact US: help@patsnap.com