Learning dataset creation method and device
A data set and guided configuration technology, applied in machine learning, image data processing, character and pattern recognition, etc., can solve problems such as inability to hide
- Summary
- Abstract
- Description
- Claims
- Application Information
AI Technical Summary
Problems solved by technology
Method used
Image
Examples
Embodiment 1
[0115] figure 1 A schematic flow of the process of creating a learning data set and a schematic flow of the process of object recognition and position and posture estimation are shown. Such as figure 1 As shown, in the stage of creating a learning data set, first, an AR marker (two-dimensional graphic marker) is used as a visual marker, and the object information of the object is associated with the AR marker (step S01). Next, prepare a jig for generating a learning data set marked with the associated two-dimensional graphics (step S02). The area of the jig for generating the learning data set is used as a guide placement object (step S03). In the state where the object is arranged, a multi-viewpoint image group of the object is acquired (step S04 ). A two-dimensional graphic mark is detected and recognized on the acquired image group (step S05). A bounding box enclosing the entire object is set to the acquired image group (step S06). Associating the estimated pose info...
Embodiment 2
[0201] Figure 19 A schematic diagram of an image used to create the learning data set of the second embodiment is shown. Such as Figure 19 As shown in FIG. 7 , the conveyor belt 12 , objects ( 5 a to 5 c ), and bounding boxes ( 6 d to 6 f ) are displayed in the image 7 a.
[0202] In this embodiment, a three-dimensional bounding box ( 6d - 6f ) is set, which can be realized by setting a plurality of AR markers 3 .
[0203] That is, in this embodiment, the learning data set is created using the boards (14a-14c), but as Figure 7 As shown, taking the center point P of the circular plate 14a 1 as the origin, such as Figure 5 As shown, the object 5 is arranged using the position adjustment guide 18a or the like so that the center of the bottom of the object becomes the origin.
[0204] The height, shape, width, and depth of the object 5 are stored as object information data in advance as object attribute information. figure 1 shown in the database 8. can be obtained from...
Embodiment 3
[0206] Figure 20 It is a plan view of the learning data set generation jig in Example 3, and the number of displayed AR markers is (1) 8 and (2) 3. Such as Figure 20 As shown in (1), eight AR marks (3a to 3h) are provided on the circular base portion 17 of the plate 140a. Additionally, if Figure 20As shown in (2), three AR marks (3a to 3c) are provided on the circular base portion 17 of the plate 140b.
[0207] Here, it is not necessary to set 12 AR markers (3a-3l) like the boards (14a-14c) of Embodiment 1, and a smaller number can be set in consideration of the type, shape, manufacturing cost, etc. of the object 5. The structure of the AR marker. However, when capturing an image, it is preferable to capture two or more AR markers. This is because, as described above, by capturing two or more AR markers, it is possible to improve the recognition accuracy of the object, and it is also possible to set a three-dimensional bounding box.
PUM
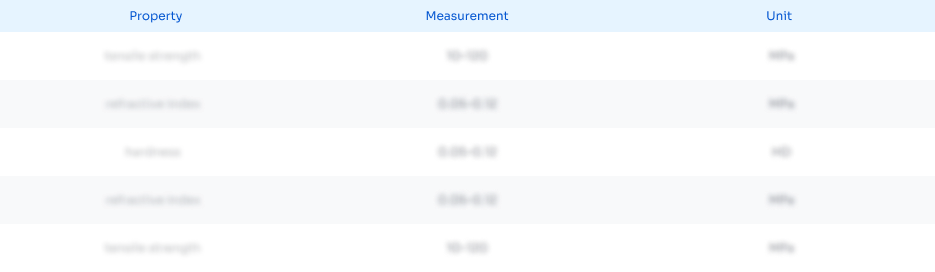
Abstract
Description
Claims
Application Information
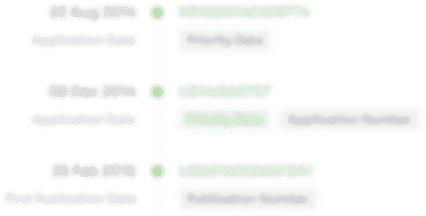
- R&D Engineer
- R&D Manager
- IP Professional
- Industry Leading Data Capabilities
- Powerful AI technology
- Patent DNA Extraction
Browse by: Latest US Patents, China's latest patents, Technical Efficacy Thesaurus, Application Domain, Technology Topic, Popular Technical Reports.
© 2024 PatSnap. All rights reserved.Legal|Privacy policy|Modern Slavery Act Transparency Statement|Sitemap|About US| Contact US: help@patsnap.com