Seismic super-resolution inversion method based on model-driven deep learning
A model-driven, deep learning technology, applied in seismology, seismic signal processing, scientific instruments, etc., can solve problems such as low signal-to-noise ratio of surface seismic data, inversion resolution and fidelity that cannot meet oil and gas exploration and development, etc. Achieve the effect of fast calculation and avoid the design of regular items
- Summary
- Abstract
- Description
- Claims
- Application Information
AI Technical Summary
Problems solved by technology
Method used
Image
Examples
Embodiment Construction
[0054] In order to better understand the present invention, the technical solutions in the embodiments of the present invention will be described in contemplation, and It is an embodiment of the invention, not all of the embodiments. Based on the embodiments in the present invention, those of ordinary skill in the art may belong to the scope of the present invention in the range of the present invention without all other embodiments obtained without making creative labor.
[0055] It should be noted that the specification and claims of the present invention and the terms "first", "second", "second", or the like are used to distinguish a similar object without having to describe a particular order or award order. It should be understood that the data such as use can be interchanged in appropriate, so that the embodiments of the invention described herein can be implemented in the order other than those illustrated or described herein. Moreover, the terms "including" and "have" and ...
PUM
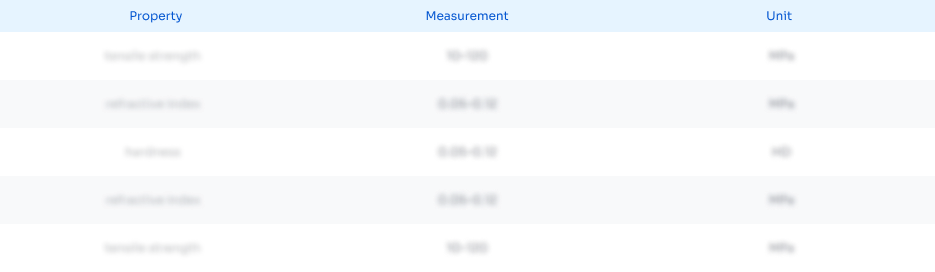
Abstract
Description
Claims
Application Information
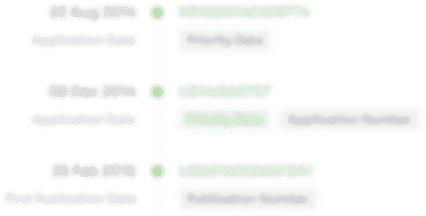
- R&D Engineer
- R&D Manager
- IP Professional
- Industry Leading Data Capabilities
- Powerful AI technology
- Patent DNA Extraction
Browse by: Latest US Patents, China's latest patents, Technical Efficacy Thesaurus, Application Domain, Technology Topic.
© 2024 PatSnap. All rights reserved.Legal|Privacy policy|Modern Slavery Act Transparency Statement|Sitemap