Method and device for optimizing FKNN model parameters based on variation Sashimi swarm algorithm
A salp group and model parameter technology, applied in the computer field, achieves the effects of high classification accuracy, strong ability, and high convergence accuracy
- Summary
- Abstract
- Description
- Claims
- Application Information
AI Technical Summary
Problems solved by technology
Method used
Image
Examples
Embodiment Construction
[0037]In order to make the objectives, technical solutions and advantages of the present invention clearer, the present invention will be further described in detail below with reference to the accompanying drawings.
[0038]Such asfigure 1As shown in the first embodiment of the present invention, a method for optimizing FKNN model parameters based on the mutant salvage swarm algorithm is proposed, and the method includes the following steps:
[0039]Step S1: obtaining sample data and normalizing the obtained sample data;
[0040]The specific process is that the sample data comes from a variety of different fields and can be designed according to actual needs, such as the medical field and the financial field. The data attribute categories are divided into data attributes and category attributes. For example, for a single sample attribute of breast cancer disease data, the data attribute value is divided into two categories, namely data attribute X1-X9Represents the relevant medical patholog...
PUM
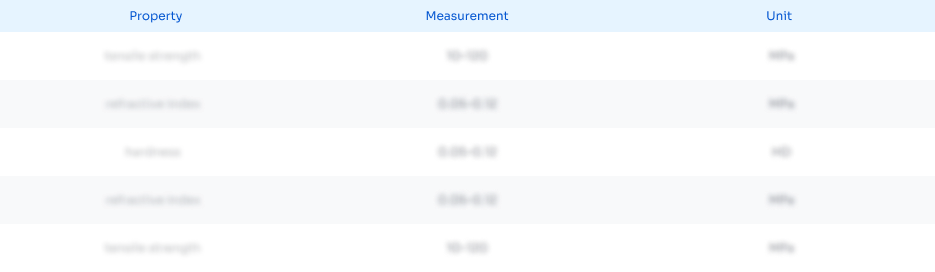
Abstract
Description
Claims
Application Information
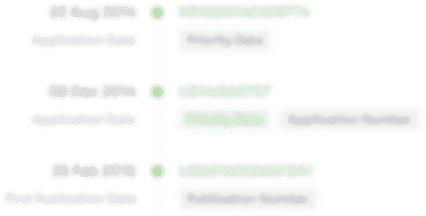
- R&D
- Intellectual Property
- Life Sciences
- Materials
- Tech Scout
- Unparalleled Data Quality
- Higher Quality Content
- 60% Fewer Hallucinations
Browse by: Latest US Patents, China's latest patents, Technical Efficacy Thesaurus, Application Domain, Technology Topic, Popular Technical Reports.
© 2025 PatSnap. All rights reserved.Legal|Privacy policy|Modern Slavery Act Transparency Statement|Sitemap|About US| Contact US: help@patsnap.com