Prisoner dangerous behavior prediction method and system based on effective influence factors
A technology of serving prisoners and influencing factors, applied in the research field of risk behavior prediction in prisons, can solve the problems of model correction and optimization lag, and achieve the effect of reducing complexity, improving efficiency and accuracy
- Summary
- Abstract
- Description
- Claims
- Application Information
AI Technical Summary
Problems solved by technology
Method used
Image
Examples
Embodiment 1
[0060] A method for predicting risky behaviors of prisoners based on effective impact factors, such as figure 2 shown, including the following steps:
[0061] (1) Structured processing:
[0062] 1) Data cleaning: Supplement, modify, and delete the default items, irregular items, and erroneous items in the characteristic information of inmates, so as to ensure the validity of the data set.
[0063] The characteristic information of inmates includes criminal basic information, crime information, psychological condition and daily behavior;
[0064] The basic information, criminal information, psychological status and daily behavior of criminals include the specific information shown in Table 1:
[0065] Table 1
[0066]
[0067] 2) extract effective impact factor: the present invention proposes a kind of hierarchical feature extraction method based on Pearson correlation coefficient method (PCC) and information gain (IG), from two aspects of correlation and information amo...
Embodiment 2
[0093] According to a method for predicting dangerous behaviors of prisoners based on effective impact factors described in Example 1, the difference is that:
[0094] Use r to represent the PCC coefficient, and its calculation formula is shown in formula (I):
[0095]
[0096] In formula (I), X i and Indicates the value of the data of each prisoner and the mean value of the data of all prisoners in the feature field in the data set after data cleaning, Y i and Represents the value of the label of each sample and the overall mean, i represents a sample under the feature, and n represents the total number of samples.
Embodiment 3
[0098] According to a method for predicting dangerous behaviors of prisoners based on effective impact factors described in Example 1, the difference is that:
[0099] Use IG(Y|X) to represent the information entropy of a feature, and its calculation formula is shown in formula (II), formula (III) and formula (IV):
[0100]
[0101]
[0102] IG(Y|X)=H(Y)-H(Y|X) (IV)
[0103] In formula (II), formula (III) and formula (IV), H(X) refers to the information entropy of feature field X, p(x i ) means that the value of this feature is x i probability of x i Indicates the value under this feature, b is the base of the logarithm, taking 2; H(Y|X) refers to the conditional entropy; H(Y) refers to the amount of information of the category label.
PUM
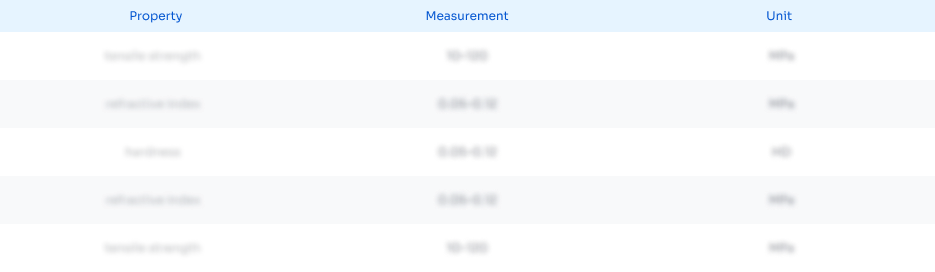
Abstract
Description
Claims
Application Information
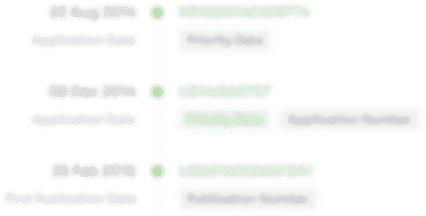
- R&D Engineer
- R&D Manager
- IP Professional
- Industry Leading Data Capabilities
- Powerful AI technology
- Patent DNA Extraction
Browse by: Latest US Patents, China's latest patents, Technical Efficacy Thesaurus, Application Domain, Technology Topic.
© 2024 PatSnap. All rights reserved.Legal|Privacy policy|Modern Slavery Act Transparency Statement|Sitemap