Unsupervised domain adaptation method and system based on target domain self-supervised learning
A target domain, supervised learning technology, applied in the unsupervised domain adaptation domain, can solve problems such as over-segmentation, neglect, and under-segmentation
- Summary
- Abstract
- Description
- Claims
- Application Information
AI Technical Summary
Problems solved by technology
Method used
Image
Examples
Embodiment Construction
[0060] The present invention will be described in detail below in conjunction with specific embodiments. The following examples will help those skilled in the art to further understand the present invention, but do not limit the present invention in any form. It should be noted that those skilled in the art can make several changes and improvements without departing from the concept of the present invention. These all belong to the protection scope of the present invention.
[0061] like figure 1 As shown, it is a flow chart of an embodiment of an unsupervised domain adaptation method based on self-supervised learning in the target domain of the present invention. The method uses a deep convolutional neural network to extract images from the source domain and the target domain through the domain-invariant feature extraction step. Extracting the domain invariant features of the image, obtaining the respective image features of the source domain and the target domain, and usi...
PUM
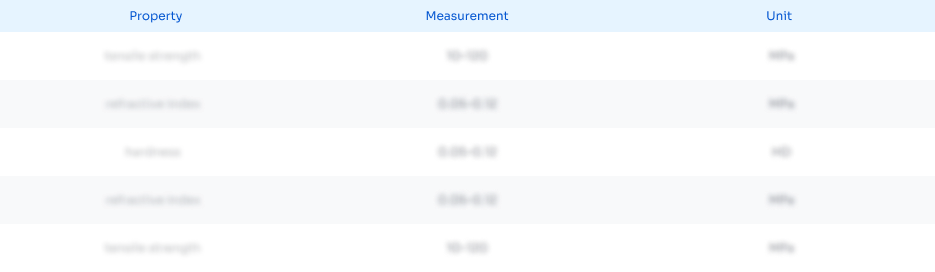
Abstract
Description
Claims
Application Information
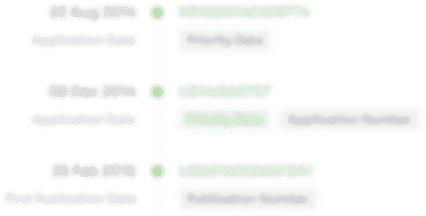
- R&D Engineer
- R&D Manager
- IP Professional
- Industry Leading Data Capabilities
- Powerful AI technology
- Patent DNA Extraction
Browse by: Latest US Patents, China's latest patents, Technical Efficacy Thesaurus, Application Domain, Technology Topic, Popular Technical Reports.
© 2024 PatSnap. All rights reserved.Legal|Privacy policy|Modern Slavery Act Transparency Statement|Sitemap|About US| Contact US: help@patsnap.com