Model compression method and system based on sparse convolutional neural network, and related equipment
A technology of convolutional neural network and compression method, which is applied in biological neural network models, neural learning methods, neural architectures, etc., can solve problems such as difficult edge equipment, large model parameters and calculations, and operation, to achieve guaranteed performance, The effect of mitigating occupancy problems
- Summary
- Abstract
- Description
- Claims
- Application Information
AI Technical Summary
Problems solved by technology
Method used
Image
Examples
Embodiment Construction
[0053] Preferred embodiments of the present invention are described below with reference to the accompanying drawings. Those skilled in the art should understand that these embodiments are only used to explain the technical principles of the present invention, and are not intended to limit the protection scope of the present invention.
[0054] figure 1 It is a schematic diagram of the main steps of the embodiment of the model compression method based on the sparse convolutional neural network of the present invention. Such as figure 1 As shown, the model compression method of this embodiment includes steps S10-S40:
[0055] In step S10, the model to be compressed is obtained by performing sparse regularization training on the model.
[0056] Specifically, a penalty factor can be added to the loss function by the method shown in formula (1):
[0057] L'=L+λR(X) (1)
[0058] Among them, L' is the loss function with the penalty factor added, L is the original loss function, λ...
PUM
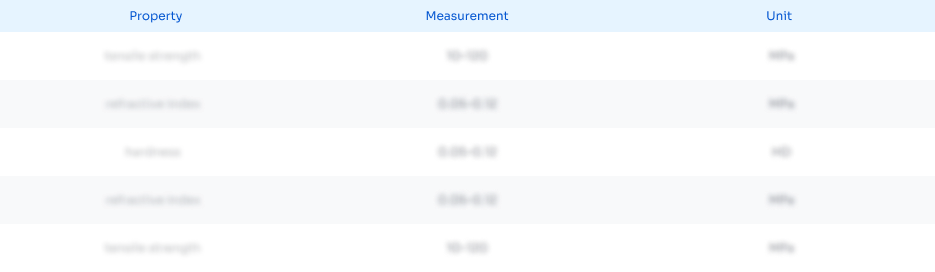
Abstract
Description
Claims
Application Information
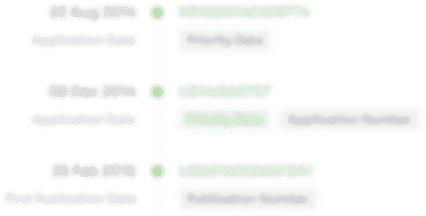
- R&D Engineer
- R&D Manager
- IP Professional
- Industry Leading Data Capabilities
- Powerful AI technology
- Patent DNA Extraction
Browse by: Latest US Patents, China's latest patents, Technical Efficacy Thesaurus, Application Domain, Technology Topic, Popular Technical Reports.
© 2024 PatSnap. All rights reserved.Legal|Privacy policy|Modern Slavery Act Transparency Statement|Sitemap|About US| Contact US: help@patsnap.com