Spine MRI image key point detection method based on deep learning
A technology of deep learning and detection methods, applied in the field of computer vision, can solve problems such as large subjective influence, poor robustness, and inapplicability to complex and changeable medical scenarios, so as to reduce the burden on doctors, avoid the influence of subjective factors, and avoid artificial The effect of labeling
- Summary
- Abstract
- Description
- Claims
- Application Information
AI Technical Summary
Problems solved by technology
Method used
Image
Examples
Embodiment Construction
[0025] The present invention will be described in detail below according to the accompanying drawings.
[0026] refer to figure 1 , the data sets of the training network in the present invention are all self-built data sets, and the key points of the spine are all marked by doctors and experts. key point. The basic process of model training is as follows:
[0027] 1. Collect spine MRI images and randomly select a part of the images as the initial data set.
[0028] 2. Label or correct the dataset, and use the labeled dataset to train the model.
[0029] 3. Use the trained model to predict the newly acquired spine MRI images and add them to the data set.
[0030] 4. Repeat steps 2 and 3 until the accuracy of the model meets the usage requirements.
[0031] figure 2 The target detection network described in is preferably YOLOv3. In this method, during the training process, the vertebrae are divided into two categories, S1 and NS1 (sacral 1 and non-sacral 1), according to ...
PUM
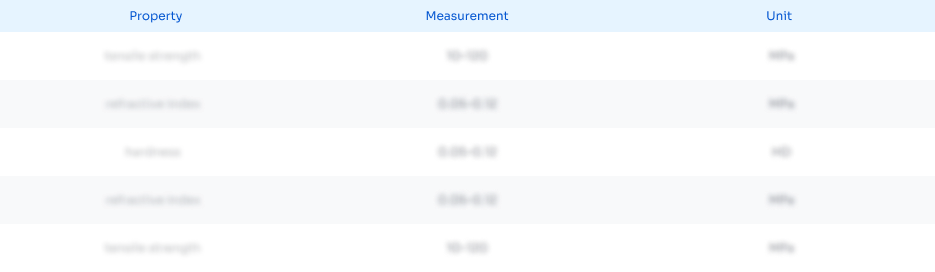
Abstract
Description
Claims
Application Information
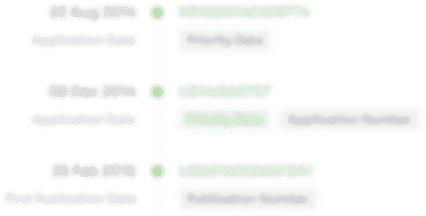
- R&D Engineer
- R&D Manager
- IP Professional
- Industry Leading Data Capabilities
- Powerful AI technology
- Patent DNA Extraction
Browse by: Latest US Patents, China's latest patents, Technical Efficacy Thesaurus, Application Domain, Technology Topic, Popular Technical Reports.
© 2024 PatSnap. All rights reserved.Legal|Privacy policy|Modern Slavery Act Transparency Statement|Sitemap|About US| Contact US: help@patsnap.com