Driver longitudinal car-following behavior model construction method based on deep reinforcement learning
A technology of reinforcement learning and construction methods, applied in the direction of neural learning methods, biological neural network models, neural architectures, etc., can solve the problems of randomness and complexity of car-following behaviors of difficult drivers, and achieve the effect of realizing reproducibility
- Summary
- Abstract
- Description
- Claims
- Application Information
AI Technical Summary
Problems solved by technology
Method used
Image
Examples
Embodiment Construction
[0038] The following embodiments will further illustrate the present invention in conjunction with the accompanying drawings.
[0039] The schematic flow chart of the driver's longitudinal car-following behavior model of the present invention is as follows: figure 1 shown. Firstly, collect the data of the driver’s car-following behavior that conforms to the characteristics of Chinese roads, and give the key parameters representing the benchmark information of the driver’s behavior. Secondly, build a deep neural network structure of the driver’s longitudinal car-following behavior model to effectively solve the problem of driver’s car-following behavior. The decision-making problem in the continuous action space in the behavior process, and then, the training method of the driver's longitudinal car-following behavior model based on deep reinforcement learning is designed to realize the verification and evaluation of the driver's longitudinal car-following behavior model. The s...
PUM
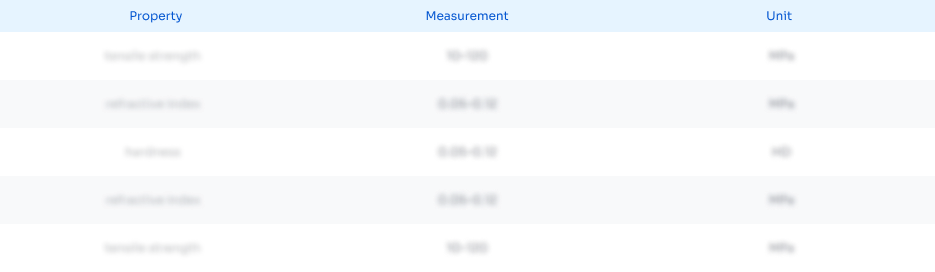
Abstract
Description
Claims
Application Information
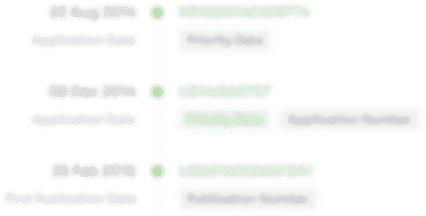
- R&D Engineer
- R&D Manager
- IP Professional
- Industry Leading Data Capabilities
- Powerful AI technology
- Patent DNA Extraction
Browse by: Latest US Patents, China's latest patents, Technical Efficacy Thesaurus, Application Domain, Technology Topic, Popular Technical Reports.
© 2024 PatSnap. All rights reserved.Legal|Privacy policy|Modern Slavery Act Transparency Statement|Sitemap|About US| Contact US: help@patsnap.com