Commodity classification method based on multi-modal deep neural network model
A technology of deep neural network and classification method, which is applied in the field of commodity classification based on multi-modal deep neural network model, can solve the problems of decision-making fusion method learning and data fusion method, which is difficult to directly combine different modal data, and achieves a small number of parameters , fast training speed and simple network structure
- Summary
- Abstract
- Description
- Claims
- Application Information
AI Technical Summary
Problems solved by technology
Method used
Image
Examples
Embodiment Construction
[0035] Embodiments of the invention are described in detail below, examples of which are illustrated in the accompanying drawings. The embodiments described below by referring to the figures are exemplary only for explaining the present invention and should not be construed as limiting the present invention.
[0036] Most of the currently proposed deep neural network models for commodity classification are based on unimodal data only. In actual situations, there are many forms of information about products, including product text, product images, videos, etc., and models based on unimodal data do not make full use of these information. Based on this requirement, the present invention proposes a commodity classification method based on a multimodal deep neural network model.
[0037] Such as figure 1 Shown, a kind of commodity classification method based on multimodal deep neural network model of the present invention comprises the following steps:
[0038] 1. First create a...
PUM
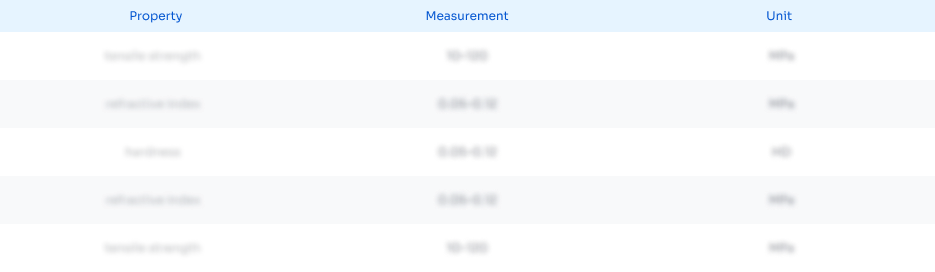
Abstract
Description
Claims
Application Information
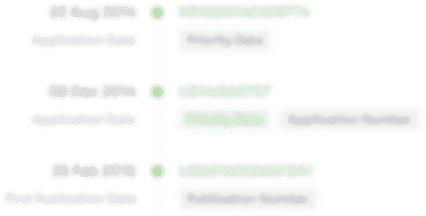
- R&D Engineer
- R&D Manager
- IP Professional
- Industry Leading Data Capabilities
- Powerful AI technology
- Patent DNA Extraction
Browse by: Latest US Patents, China's latest patents, Technical Efficacy Thesaurus, Application Domain, Technology Topic, Popular Technical Reports.
© 2024 PatSnap. All rights reserved.Legal|Privacy policy|Modern Slavery Act Transparency Statement|Sitemap|About US| Contact US: help@patsnap.com