Semi-supervised learning method and system based on target segmentation field self-learning
A semi-supervised learning and target segmentation technology, applied in the field of computer vision and image processing, can solve the problems of reduced model performance, inaccurate confidence map, and inability to pay attention to whether the segmentation target is accurate, etc., to increase quality assessment and optimization, and accurate quality assessment , the effect of increasing accuracy
- Summary
- Abstract
- Description
- Claims
- Application Information
AI Technical Summary
Problems solved by technology
Method used
Image
Examples
Embodiment Construction
[0065] The present invention will be described in detail below in conjunction with specific embodiments. The following examples will help those skilled in the art to further understand the present invention, but do not limit the present invention in any form. It should be noted that those skilled in the art can make several modifications and improvements without departing from the concept of the present invention. These all belong to the protection scope of the present invention.
[0066] figure 1 It is a flow chart of a semi-supervised learning method based on self-learning of target segmentation domain provided by an embodiment of the present invention.
[0067] like figure 1 As shown, the semi-supervised learning method based on target segmentation field self-learning provided by this embodiment may include the following steps:
[0068] S100, using the labeled data in the training data set to train the initial segmentation network;
[0069] S200, generating pseudo-labe...
PUM
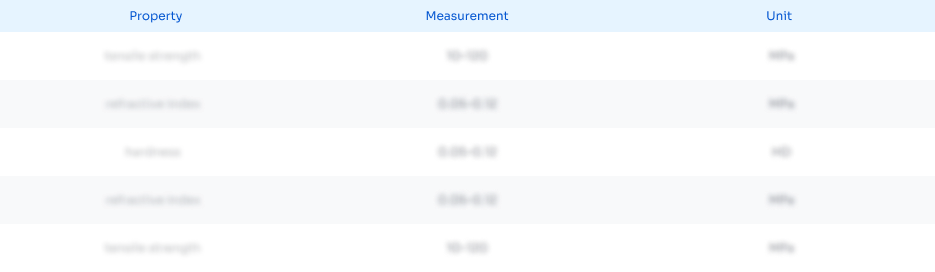
Abstract
Description
Claims
Application Information
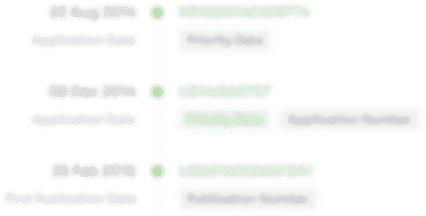
- R&D Engineer
- R&D Manager
- IP Professional
- Industry Leading Data Capabilities
- Powerful AI technology
- Patent DNA Extraction
Browse by: Latest US Patents, China's latest patents, Technical Efficacy Thesaurus, Application Domain, Technology Topic, Popular Technical Reports.
© 2024 PatSnap. All rights reserved.Legal|Privacy policy|Modern Slavery Act Transparency Statement|Sitemap|About US| Contact US: help@patsnap.com