Oil reservoir geologic modeling static parameter distribution prediction method based on neighbor neural network
A neural network model and neural network technology, applied in the field of reservoir geological modeling space interpolation, can solve the problems of large uncertainty and low interpolation precision, and achieve the effect of quantifying uncertainty and improving precision
- Summary
- Abstract
- Description
- Claims
- Application Information
AI Technical Summary
Problems solved by technology
Method used
Image
Examples
Embodiment Construction
[0039] The present invention will be described in further detail below.
[0040] The present invention firstly selects the oil reservoir area to be predicted, then retrieves the known spatial coordinates and corresponding static parameter values of all wells within the geological prediction range of the oil reservoir, and assumes that there are wells in the middle and late stages of development within the range N wells, and then use the nearest neighbor algorithm to find m adjacent wells for each well. Then a random layer ò is added before any layer after the input layer of the existing neural network to obtain a neural network model. The known spatial coordinates of the i-th well, the known spatial coordinates of the m adjacent wells corresponding to the i-th well, and the known static parameters of the m adjacent wells corresponding to the i-th well are used as the i-th sample, i= 1, 2, 3...N, all samples constitute a data set; 90% of the samples in the data set are rando...
PUM
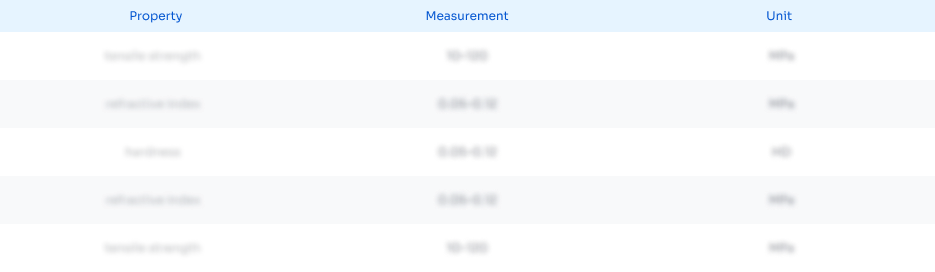
Abstract
Description
Claims
Application Information
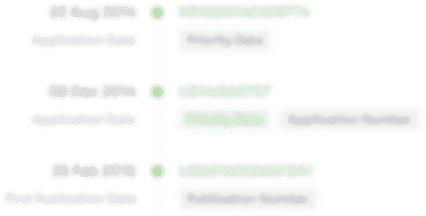
- R&D Engineer
- R&D Manager
- IP Professional
- Industry Leading Data Capabilities
- Powerful AI technology
- Patent DNA Extraction
Browse by: Latest US Patents, China's latest patents, Technical Efficacy Thesaurus, Application Domain, Technology Topic, Popular Technical Reports.
© 2024 PatSnap. All rights reserved.Legal|Privacy policy|Modern Slavery Act Transparency Statement|Sitemap|About US| Contact US: help@patsnap.com