Personalized recommendation method based on knowledge graph convolution algorithm
A knowledge map and algorithm technology, applied in the field of hybrid recommendation system, can solve the problems of simplicity, inaccurate entity and relationship vector representation, etc., and achieve the effect of accurate semantic structure information, accurate description of interests, and enhanced interpretability
- Summary
- Abstract
- Description
- Claims
- Application Information
AI Technical Summary
Problems solved by technology
Method used
Image
Examples
Embodiment Construction
[0039] The technical scheme of the present invention will be further described in detail below in conjunction with the accompanying drawings.
[0040] Such as figure 1 and figure 2 As shown, a personalized recommendation method based on the knowledge graph convolution algorithm, the method learns its high-order feature representation by polymerizing the multi-hop receptive field information of the entity embedding vector in the domain knowledge graph; the method first constructs each item In the domain knowledge map, the entity receptive field set from 1 to d jumps, and then the embedding vector of the receptive field entity from d to 1 jump is aggregated by the graph convolution algorithm, and finally the item embedding vector containing high-order neighbor information is calculated; during the aggregation process, the Based on the graph convolution algorithm, the knowledge map representation learning model DistMult and the attention mechanism representing the user interest...
PUM
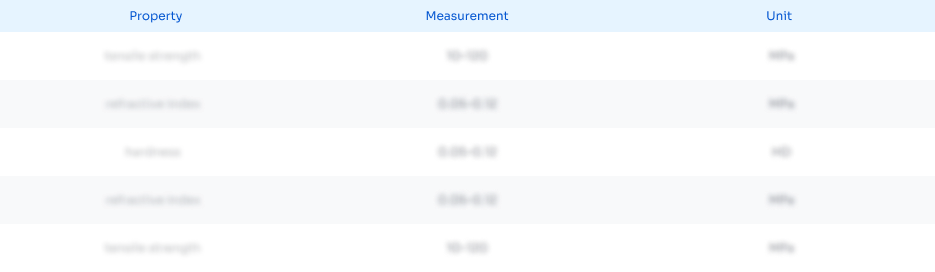
Abstract
Description
Claims
Application Information
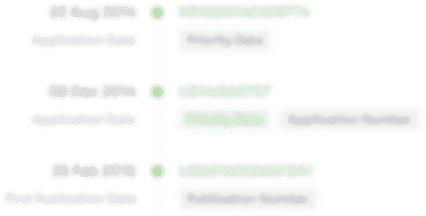
- R&D Engineer
- R&D Manager
- IP Professional
- Industry Leading Data Capabilities
- Powerful AI technology
- Patent DNA Extraction
Browse by: Latest US Patents, China's latest patents, Technical Efficacy Thesaurus, Application Domain, Technology Topic, Popular Technical Reports.
© 2024 PatSnap. All rights reserved.Legal|Privacy policy|Modern Slavery Act Transparency Statement|Sitemap|About US| Contact US: help@patsnap.com