Efficient contribution evaluation method in federated learning scene
A federated and contributed technology, applied in the field of machine learning and data analysis, can solve problems that are impossible to implement and cannot directly use federated systems, and achieve the effects of improving performance, speeding up model convergence, and speeding up convergence
- Summary
- Abstract
- Description
- Claims
- Application Information
AI Technical Summary
Problems solved by technology
Method used
Image
Examples
Embodiment Construction
[0012] The present invention will be described in detail below in conjunction with:
[0013] In horizontal federated learning, participating users download the latest global model from the server, and then use local data to train the local model and upload it to the server; the server aggregates the local models uploaded by each user to update the global model. In the federated learning system, in order to protect data privacy, the server cannot directly access the user's training data, so the server uses the training log (local model uploaded by the user) to evaluate the weight of each user in gradient aggregation, and then calculates the weight of each user based on the weight. Model contribution. In this module, the main steps are as follows:
[0014] 1) Calculate the weight based on the training log: the server uses the training log of federated learning (the user's model update parameters t∈[T], n∈[N], t represents the number of training rounds, and n represents the nu...
PUM
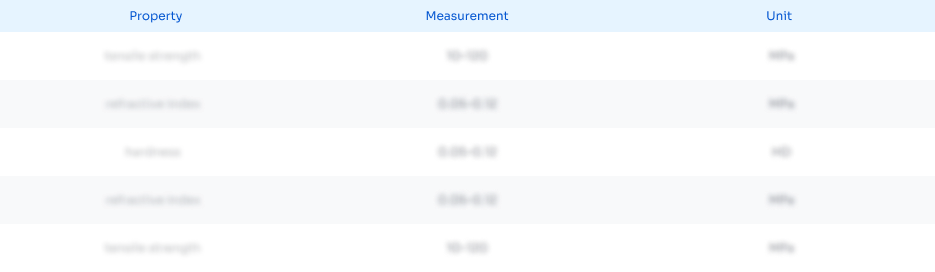
Abstract
Description
Claims
Application Information
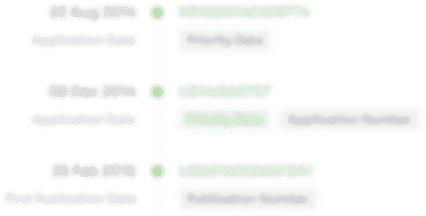
- R&D Engineer
- R&D Manager
- IP Professional
- Industry Leading Data Capabilities
- Powerful AI technology
- Patent DNA Extraction
Browse by: Latest US Patents, China's latest patents, Technical Efficacy Thesaurus, Application Domain, Technology Topic.
© 2024 PatSnap. All rights reserved.Legal|Privacy policy|Modern Slavery Act Transparency Statement|Sitemap