A Multimodal Dynamic Gesture Recognition Method Based on Lightweight 3D Residual Network and TCN
A dynamic gesture and recognition method technology, applied in character and pattern recognition, biological neural network model, neural learning method, etc., can solve the problem of high complexity of the method
- Summary
- Abstract
- Description
- Claims
- Application Information
AI Technical Summary
Problems solved by technology
Method used
Image
Examples
Embodiment Construction
[0044] The technical solutions in the embodiments of the present invention will be described clearly and in detail below with reference to the accompanying drawings in the embodiments of the present invention. The described embodiments are only some of the embodiments of the invention.
[0045] The technical scheme that the present invention solves the above-mentioned technical problems is:
[0046] like figure 1 As shown, a multi-modal dynamic gesture recognition method based on a lightweight 3D residual network and TCN provided by this embodiment includes the following steps:
[0047] Step 1: According to the frame rate of the gesture video in the original data set, sample each gesture video, generate a number of pictures corresponding to the frame rate of the video, and save the pictures in chronological order. In order to ensure that the input data has the same dimension, the window sliding method is used to set the input reference frame number of each gesture video. It...
PUM
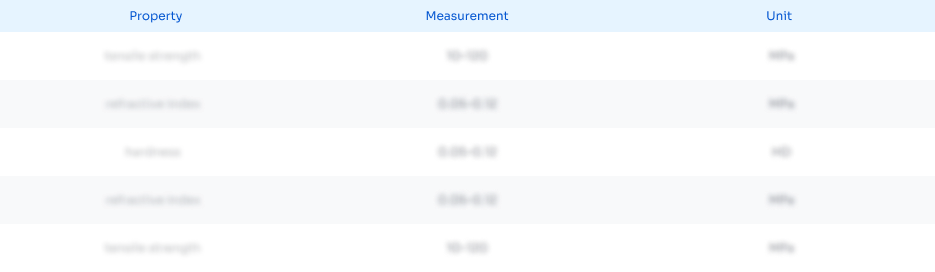
Abstract
Description
Claims
Application Information
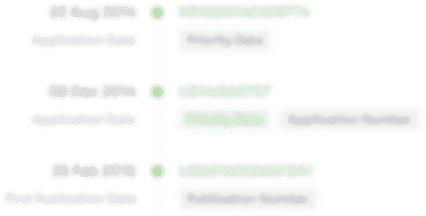
- R&D Engineer
- R&D Manager
- IP Professional
- Industry Leading Data Capabilities
- Powerful AI technology
- Patent DNA Extraction
Browse by: Latest US Patents, China's latest patents, Technical Efficacy Thesaurus, Application Domain, Technology Topic, Popular Technical Reports.
© 2024 PatSnap. All rights reserved.Legal|Privacy policy|Modern Slavery Act Transparency Statement|Sitemap|About US| Contact US: help@patsnap.com