A Deep Reinforcement Learning Approach for Continuous Coverage of Specific Areas by Multi-UAV Systems
A multi-UAV and reinforcement learning technology, applied in the field of deep reinforcement learning, can solve the problem of drone coordination without considering the continuous coverage of the area, without dealing with the control problem of heterogeneous drones, and without minimizing the cycle of continuous coverage and other issues to achieve the effect of minimizing the coverage period, increasing the convergence speed, and improving the coverage performance
- Summary
- Abstract
- Description
- Claims
- Application Information
AI Technical Summary
Problems solved by technology
Method used
Image
Examples
Embodiment Construction
[0044] The present invention will be further described in detail below with reference to the accompanying drawings and specific embodiments.
[0045] like figure 1 As shown, the deep reinforcement learning method for continuous coverage of a specific area by a multi-UAV system of the present invention includes the following steps:
[0046] Step S1: establish a deep convolutional neural network model for feature extraction of the area to be covered;
[0047]By using the convolutional neural network to extract the features of the area to be covered, the spatial structure information of the area can be effectively used, and the coverage performance can be improved; by using the local perception characteristics of the convolutional neural network, local coordinated communication between UAVs can be realized; The weight sharing feature of convolutional neural network greatly reduces model parameters, thereby improving the convergence speed of model training.
[0048] In step S1, ...
PUM
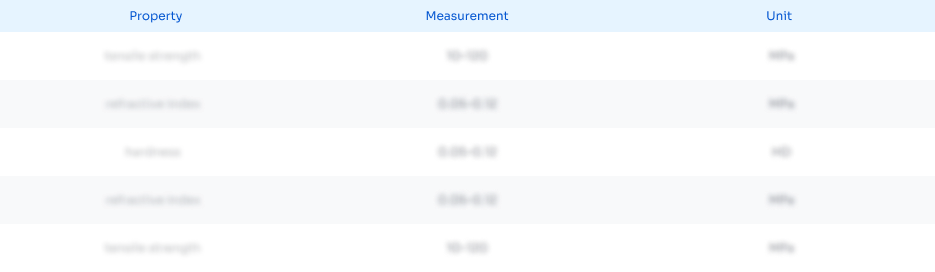
Abstract
Description
Claims
Application Information
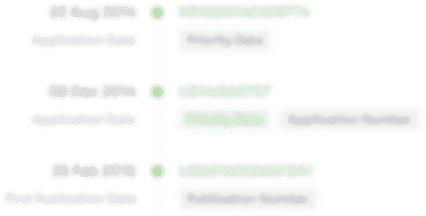
- R&D Engineer
- R&D Manager
- IP Professional
- Industry Leading Data Capabilities
- Powerful AI technology
- Patent DNA Extraction
Browse by: Latest US Patents, China's latest patents, Technical Efficacy Thesaurus, Application Domain, Technology Topic, Popular Technical Reports.
© 2024 PatSnap. All rights reserved.Legal|Privacy policy|Modern Slavery Act Transparency Statement|Sitemap|About US| Contact US: help@patsnap.com