A Hyperspectral Image Classification Method Extracted from Local to Global Context Information
An information extraction and image classification technology, applied in the field of remote sensing image processing, can solve problems such as time-consuming, high computational cost, and difficulty in determining the size of spatial blocks, and achieve the effect of improving isolated and misclassified areas.
- Summary
- Abstract
- Description
- Claims
- Application Information
AI Technical Summary
Problems solved by technology
Method used
Image
Examples
Embodiment 1
[0057] The present invention provides a hyperspectral image classification method for extracting information from local to global context, comprising the following steps:
[0058] Step 1, enter an image to be classified, WHU-Hi-HongHu, such as Figure 1 as shown, mirror its spatial dimensions to be filled in multiples of 8.
[0059] Step 2, perform channel dimensionality reduction on the image-filled image, which further includes:
[0060] The image X after the image is filled with images is reduced by a network structure of "convolutional layer-group normalization layer-nonlinear activation layer", and the characteristic map F is output, where group normalization considers the spectral continuity of hyperspectral imagery.
[0061] Step 3.Use the Local Attention Module for local contextual information extraction, such as Figure 2 as shown. This step further includes:
[0062] Step 3.1, after the channel in step 2 is reduced, the feature map F is obtained, and the feature map F is inp...
PUM
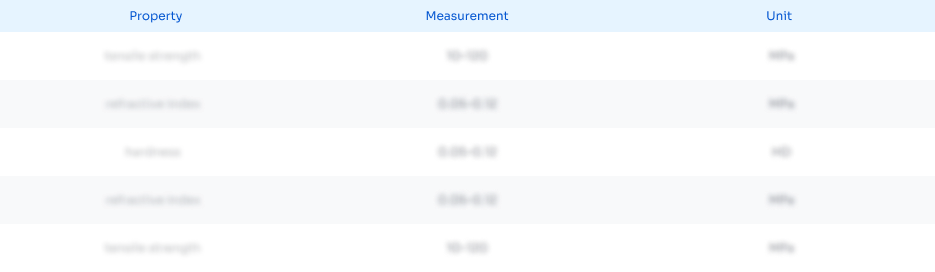
Abstract
Description
Claims
Application Information
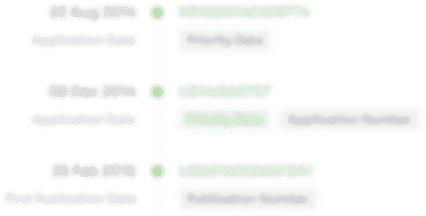
- R&D Engineer
- R&D Manager
- IP Professional
- Industry Leading Data Capabilities
- Powerful AI technology
- Patent DNA Extraction
Browse by: Latest US Patents, China's latest patents, Technical Efficacy Thesaurus, Application Domain, Technology Topic, Popular Technical Reports.
© 2024 PatSnap. All rights reserved.Legal|Privacy policy|Modern Slavery Act Transparency Statement|Sitemap|About US| Contact US: help@patsnap.com