Gas turbine abnormal state monitoring method based on frequency spectrum reconstruction errors
A gas turbine, reconstruction error technology, applied in gas turbine engine testing, jet engine testing and other directions, can solve the problems of hard learning, large span, multiple computing and storage resources, etc., to reduce training difficulty, improve reconstruction effect, and strengthen The effect of generalization performance
- Summary
- Abstract
- Description
- Claims
- Application Information
AI Technical Summary
Problems solved by technology
Method used
Image
Examples
Embodiment Construction
[0041] The present invention is further described below in conjunction with embodiment. The scope of the present invention is not limited by these Examples. The content of the present invention will be further described in detail in conjunction with the accompanying drawings for the specific working principle of the present invention.
[0042] The improved version of the deep variational autoencoder neural network model designed by the present invention is applied to an actual engineering gas turbine blade failure case, and the overall process of the method is as follows figure 1 shown.
[0043] Firstly, a wide-band acceleration sensor capable of collecting more than 20kHz is installed on the gas turbine, and the waveform signal is continuously collected from the healthy operation of the gas turbine. The 0-20kHz broadband acceleration spectrum of the collected waveform signal after Fourier transform is as follows: figure 2 shown.
[0044] When the present invention is app...
PUM
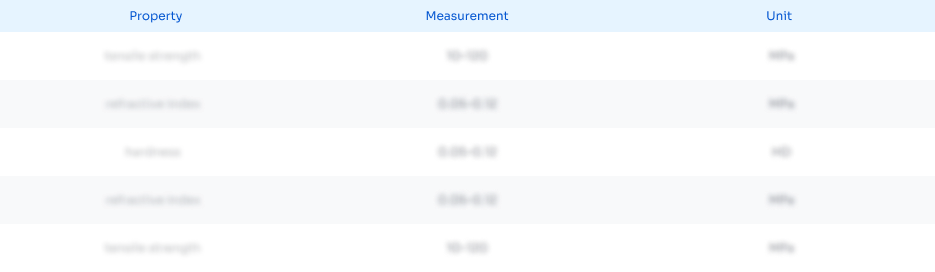
Abstract
Description
Claims
Application Information
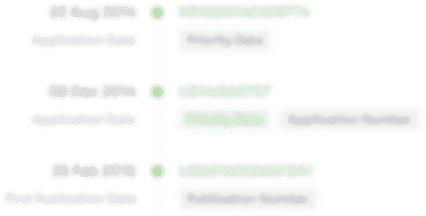
- Generate Ideas
- Intellectual Property
- Life Sciences
- Materials
- Tech Scout
- Unparalleled Data Quality
- Higher Quality Content
- 60% Fewer Hallucinations
Browse by: Latest US Patents, China's latest patents, Technical Efficacy Thesaurus, Application Domain, Technology Topic, Popular Technical Reports.
© 2025 PatSnap. All rights reserved.Legal|Privacy policy|Modern Slavery Act Transparency Statement|Sitemap|About US| Contact US: help@patsnap.com