Twin classifier certainty maximization method for cross-domain complex vision task
A classifier and deterministic technology, applied in the field of transfer learning, can solve the problems of single adaptation scene and insufficient discriminability, and achieve the effect of improving model performance, ensuring discriminability and predicting diversity.
- Summary
- Abstract
- Description
- Claims
- Application Information
AI Technical Summary
Problems solved by technology
Method used
Image
Examples
Embodiment Construction
[0040] In order to make the purpose, technical solutions and advantages of the present invention clearer, specific examples of the method of the present invention will be further described in detail.
[0041] For ease of understanding, in this example, include a source domain with the label where: n s is the sample size, is the i-th sample in the source domain, is the corresponding label, and an unlabeled target domain where: n t is the number of samples in the target domain, is the i-th sample in the target domain; the goal of the method of the present invention is to migrate the deep neural network model trained on the source domain samples to the target domain, and enable it to learn a good transferable Feature representation of sex and discriminability, so as to achieve good performance of the model on the target domain, that is, φ:X t →Y t ; The model framework of the method of the present invention comprises a feature generator G and two twin classifiers C ...
PUM
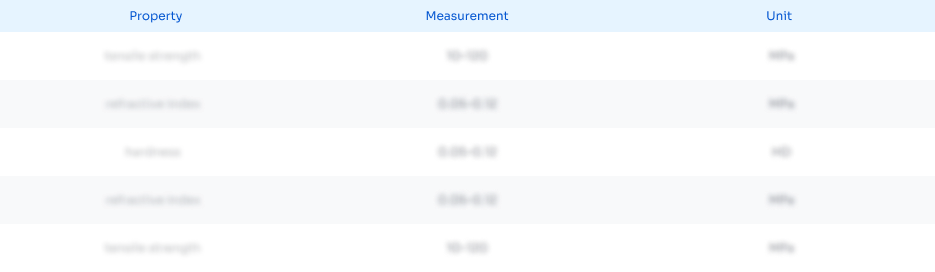
Abstract
Description
Claims
Application Information
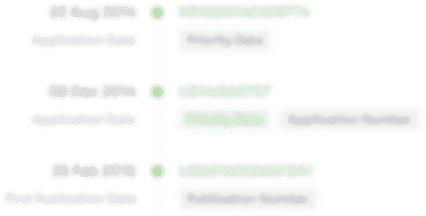
- R&D Engineer
- R&D Manager
- IP Professional
- Industry Leading Data Capabilities
- Powerful AI technology
- Patent DNA Extraction
Browse by: Latest US Patents, China's latest patents, Technical Efficacy Thesaurus, Application Domain, Technology Topic, Popular Technical Reports.
© 2024 PatSnap. All rights reserved.Legal|Privacy policy|Modern Slavery Act Transparency Statement|Sitemap|About US| Contact US: help@patsnap.com